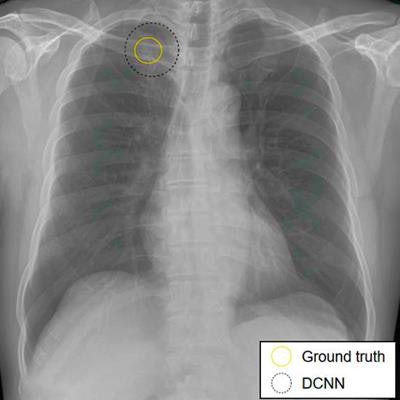
Radiologists using a deep-learning algorithm can detect more cases of lung cancer on chest radiographs than they could without help from the software, while also having fewer false positives, according to research published online November 12 in Radiology.
A team of researchers led by Dr. Byoung Wook Choi of Yonsei University Health System assessed the performance of commercially available deep convolutional neural network (DCNN)-based software in a multicenter retrospective study involving 12 radiologists. They found that radiologist sensitivity improved by an average of 5.2 percentage points after they rereviewed the x-rays with help from the software. The radiologists also had fewer false positives.
"Computer-aided detection software to detect lung nodules has not been widely accepted and utilized because of high false-positive rates, even though it provides relatively high sensitivity," Choi noted in a statement by the RSNA. "DCNN may be a solution to reduce the number of false positives."
Lung nodules have traditionally been challenging to detect on chest x-rays due to their size, density, and location. Artificial intelligence methods such as DCNNs have shown promise, however, for helping to improve detection, according to the researchers.
To evaluate the impact of the software, the researchers gathered a random selection of 800 x-rays from four participating centers: Freiburg University Hospital in Freiburg, Germany; Massachusetts General Hospital in Boston; Samsung Medical Center in Seoul, South Korea; and Severance Hospital in Seoul.
Of these 800 studies, 200 were normal exams and 600 had at least one malignant lung nodule that was confirmed by either CT imaging or pathologic exams. A total of 704 malignant nodules were confirmed; 78.6% were primary lung cancers and 21.4% were metastases. Most (56.1%) of the nodules were between 1 cm and 2 cm, with the remainder between 2 cm and 3 cm.
Next, three different radiologists from each institution independently read the chest x-rays both with and without help from the DCNN software. Of the 12 radiologists, three were resident radiologists, four were chest radiologists with five years of experience, and five were chest radiologists with more than 10 years of experience.
The radiologists then rereviewed the same exams with help from Samsung Auto Lung Nodule Detection software (Samsung Electronics). The study was funded by Samsung Electronics.
Algorithm average performance for detecting malignant lung nodules | ||
Radiologists without deep-learning software | Radiologists with deep-learning software | |
Sensitivity | 65.1% | 70.3% |
False positives per x-ray | 0.2 | 0.18 |
Approximately 15% of the nodules were detected by the radiologists only when they rereviewed the exams with the assistance of the software, the researchers noted. They also observed that the deep-learning software improved detection performance at all three experience levels for the chest radiologists in the study.
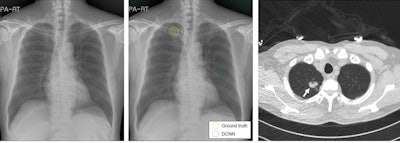
Furthermore, the software's detection performance on its own was similar to the average performance of radiologists for malignant lung nodules, according to the authors.