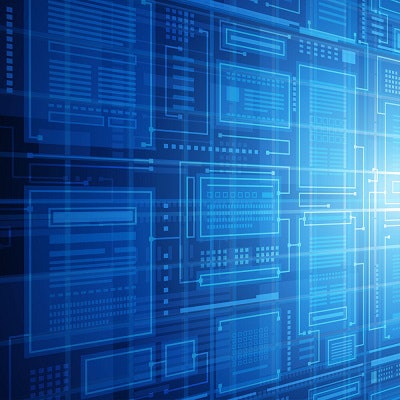
Employing multiple artificial intelligence (AI) algorithms to attack a task together results in better performance compared with using a single AI algorithm on its own, according to a study published online November 20 in Radiology: Artificial Intelligence.
Called ensemble learning, the technique is designed to combine the best aspects of multiple machine learning algorithms into a single model to perform a task, such as identifying suspicious lung lesions or estimating bone age based on skeletal x-rays. Ensemble learning works best when each of the models performs well in their own right and correlation between the predictions of each model is relatively low.
To test ensemble learning, a research team led by medical student Ian Pan of the Warren Alpert Medical School at Brown University in Providence, RI, started with 48 algorithms that were submitted as part of the RSNA's pediatric bone age challenge competition at RSNA 2017.
They then used combinations of up to 10 algorithms at a time that were trained on a dataset of over 12,000 pediatric hand x-rays in which radiologists had determined true bone age. Then, the ensemble combinations were used to analyze 200 x-rays, with the combined model producing a mean absolute deviation (MAD) of bone age, with higher deviation indicating less accuracy.
The group found that a single algorithm had a mean absolution deviation of 4.55 months, while the best-performing ensemble -- consisting of four algorithms -- had a MAD of 3.79 months.
Pan et al noted that the findings could be important as artificial intelligence begins to transition from research environments to clinical settings. Clinicians might benefit from using different AI algorithms on the same task, much like having multiple radiologists interpret an image.
The researchers also believe that competitions like the 2017 bone age challenge are important because they provide a standardized use case, a common training set, and an objective assessment method that can be applied equally to all models.
"Machine learning competitions within radiology should be encouraged to spur development of heterogeneous models whose predictions can be combined to achieve optimal performance," Pan noted.