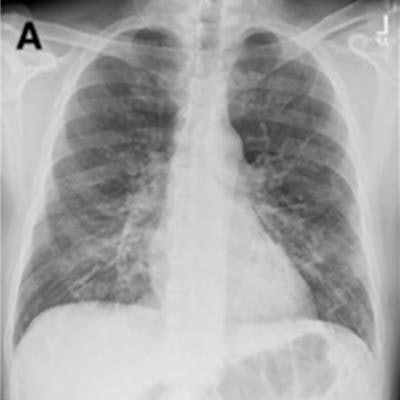
A single outpatient chest x-ray may be all it takes for an artificial intelligence (AI) algorithm to tell physicians which COVID-19 patients will likely need hospitalization and supplemental oxygen, according to a study published online May 21 in Academic Medicine.
Researchers created an AI program that first identified comorbid conditions such as cardiac arrhythmias and chronic obstructive pulmonary disease (COPD) on frontal chest x-rays of patients with COVID-19. The algorithm then predicted the likelihood of whether those patients would require full hospital admission and supplemental oxygen within 14 days.
"This deep-learning technique adds value when assessing patients with unknown medical history or awaiting laboratory testing," wrote lead author Dr. Ayis Pyrros of DuPage Medical Group in Tinley Park, Illinois.
Previous studies have shown that comorbid conditions like diabetes, cardiac arrhythmias, and COPD are associated with more severe cases of COVID-19. However, current methods for recognizing these comorbidities in ambulatory patients, such as review of manual or electronic health records or contemporaneous patient history are imperfect and often incomplete, according to the authors.
In this study, Pyrros and colleagues from the University of Illinois created a deep-learning program to predict the presence of specific comorbidities on outpatient frontal chest x-rays and tested it to see if it could help physicians identify patients who would require full hospitalization and supplemental oxygen. They included 413 patients who had received both a chest x-ray and a positive COVID-19 test in an ambulatory or immediate care setting between March 17, 2020, and October 24, 2020.
The model was trained to identify comorbidities on x-rays corresponding to a specific subset of hierarchical condition category codes from the International Classification of Diseases, Tenth Revision: diabetes with chronic complications, morbid obesity, congestive heart failure (CHF), specified heart arrhythmias, vascular disease, and COPD.
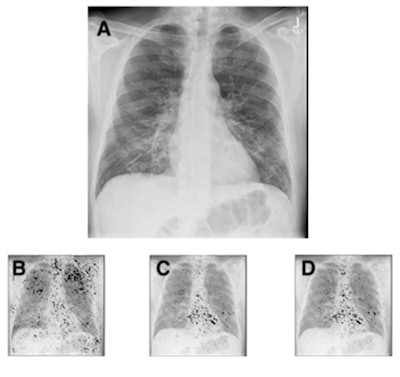
Fifty-one (12.3%) of the patients had a full hospital admission, with all requiring supplemental oxygen. Four patients died. By combining data on comorbidities found on frontal chest x-rays, as well as adding patient age, the deep-learning model predicted prolonged hospitalization and supplemental oxygenation in ambulatory COVID-19 patients with an ROC AUC of 0.837 (95%, confidence interval: 0.791-0.883).
Diabetes with chronic complications, cardiac arrhythmias, CHF, COPD, predicted age, and geographic extent and severity of opacity were all significant predictors (p < 0.05), while morbid obesity was not.
"In this preliminary study we developed an ensemble deep-learning model to predict supplemental oxygenation and hospitalization of > 2 days in outpatients testing positive for COVID-19," the researchers wrote.
No portable radiographs were used in the training or testing of the model, however, which could limit the model's use in emergency departments and hospitals, the authors stated. Moreover, implementing AI models remains a technical challenge at most institutions and practices, with relatively few available platforms or widespread adoption.
Nonetheless, the model is important because it is among the first to directly predict or quantify comorbidities on frontal chest x-rays that contribute to COVID-19 patient outcomes, they added.
"This result suggests that further validation and extension of this particular methodology is warranted," the researchers concluded.