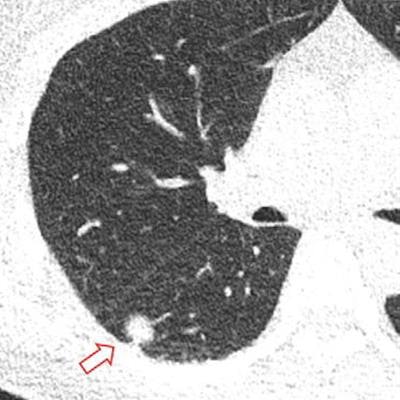
A commercially available artificial intelligence (AI)-based algorithm could help radiologists detect lung nodules on chest x-rays in patients undergoing routine health checkups, according to a study published February 7 in Radiology.
A group led by Dr. Ju Gang Nam, PhD, of Seoul National University Hospital in Korea, simulated a real-world setting by embedding a CAD algorithm into their hospital's health screening workflow. With the aid of the algorithm's analysis, radiologists doubled the number of nodules that were identified as needing follow-up.
The strength of the study is in its real-world setting, the authors wrote.
"As our trial was conducted with a pragmatic approach, almost all enrolled participants were included, which is a real clinical setting," said co-author Dr. Jin Mo Goo, PhD, in a news release from RSNA.
The researchers enrolled 10,476 adult participants who underwent at least one chest x-ray as part of a routine checkup at their hospital's health screening center between July 2020 and December 2021. Half of the images (5,238) were automatically assessed by the Insight CXR algorithm (Lunit) for the possibility of major thoracic abnormalities (pulmonary nodule, pneumonia, and pneumothorax) on a percentage scale from 0% to 100%. During image processing, the suspicious nodules were localized on the images with overlaid heat maps. The other half of the images served as controls.
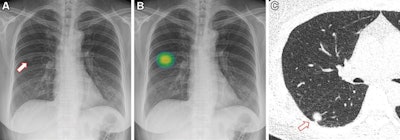
One of three radiologists with 13 to 36 years of experience interpreted each radiograph for actionable nodules with and without the AI-based CAD results. Actionable nodules were defined as solid nodules larger than eight millimeters or subsolid nodules with a solid portion larger than 6 millimeters (Lung-RADS, category 4).
The trial met its primary outcome, the authors wrote. Specifically, the detection rate of actionable nodules in the AI-assisted readers was 0.59% (31 of 5,238 participants), compared with 0.25% (13 of 5,238 participants) in the non-AI-assisted group.
Moreover, the detection rate for malignant lung nodules was higher in the AI group (.15% [8 of 5,238 participants]) compared with the non-AI group (0% [0 of 5238 participants]), the authors added.
"In health checkup participants, artificial intelligence-based software improved the detection of actionable lung nodules on chest radiographs," the group wrote.
In an accompanying editorial, Dr. William Auffermann, PhD, section chief of cardiothoracic imaging at the University of Utah medical school, wrote that the study provides much-needed randomized controlled trial data.
Many AI-based CAD software studies are performed outside clinical practice and validate algorithms using an enriched number of abnormal cases. In some studies, this percentage is near 50% of the total cases. In this study, the percentage of chest radiographs with actionable nodules was 1.1%, Auffermann noted.
Also, an increased number of abnormal cases in a nodule detection study may alter reading behavior and the threshold a radiologist uses to mark a case as concerning for nodules, he added. Thus, it is difficult to determine how the interpretive behavior of radiologists who know they are reading for a study with an enriched data set will translate to performance in routine clinical practice, he wrote.
"This study offers new data to answer this question," Auffermann concluded.