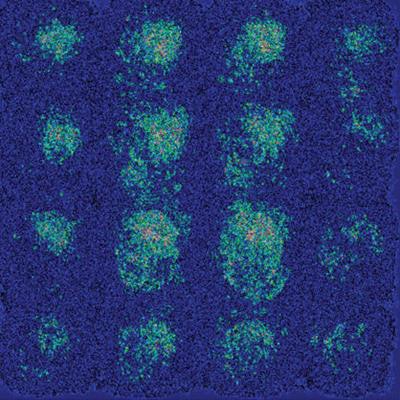
By learning to identify the metabolic patterns of Alzheimer's disease, a deep-learning algorithm for brain FDG-PET studies can accurately predict the onset of this devastating disease years before a clinical diagnosis can be made, according to new research published online in Radiology.
A team of researchers from the University of California, San Francisco (UCSF); the University of California, Berkeley; and the University of California, Davis developed a convolutional neural network (CNN) that was able to predict all cases of Alzheimer's disease in a small test of 40 cases. What's more, the algorithm outperformed interpretations by three experienced nuclear medicine physicians.
"We expect that this algorithm can be used to extract as much diagnostic information for Alzheimer's disease from FDG-PET scans as possible, in conjunction with biochemical test data and clinical symptoms, to arrive at an early prediction of Alzheimer's disease at higher accuracy than before," said co-author Dr. Jae Ho Sohn from UCSF.
Diagnosing Alzheimer's earlier
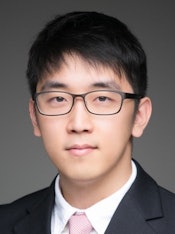
Alzheimer's disease is ultimately a clinical diagnosis, but there's a growing consensus that biochemical and imaging tests could help make this diagnosis earlier and more accurately, according to Sohn. If a diagnosis can be made before the onset of full clinical symptoms, there's an opportunity for therapeutic intervention before most of the brain tissue is atrophied.
"Unfortunately, imaging appearance of Alzheimer's disease at an early stage can be very tricky because imaging features tend to be very subtle and diffuse throughout the brain," Sohn told AuntMinnie.com.
Furthermore, there isn't a universally accepted gold-standard imaging/biochemical diagnosis for early-stage detection of Alzheimer's disease, he added.
Sohn said that his faculty advisors Dr. Benjamin Franc and Dr. Youngho Seo, as well as multiple other co-authors, became interested in using deep learning to tackle this problem. First author and student engineer Yiming Ding from the University of California, Berkeley, as well as Sohn and others in the institution's Big Data in Radiology (BDRAD) research group, took on the challenge by developing a deep-learning algorithm and validating it on clinical data.
They used data from the Alzheimer's Disease Neuroimaging Initiative (ADNI) dataset to develop a CNN with InceptionV3 architecture to predict Alzheimer's disease. Of the 2,100 FDG-PET brain images from 1,002 patients, 90% were used for training and 10% were set aside for validation.
Next, they evaluated the algorithm in an independent clinical test of 40 cases that the algorithm hadn't seen yet. The set included FDG-PET images acquired as dedicated PET-only images on an ECAT HR+ (Siemens Healthineers) scanner or as part of a PET/CT exam on a Discovery VCT (GE Healthcare) or Biograph 16 (Siemens) PET/CT scanner. The 40 cases included seven patients who were clinically diagnosed as having Alzheimer's disease, seven who had mild cognitive impairment, and 26 who had neither disease at the end of the follow-up period.
Comparison with radiology readers
Three board-certified nuclear medicine physicians -- with 36, 14, and five years of experience, respectively -- also independently read all 40 exams consecutively, performing qualitative interpretation as well as semiquantitative regional metabolic analysis using a clinical neuroanalysis software package (MIM Software). If any of the three physicians disagreed on the qualitative interpretation, then the imaging study was read by two additional nuclear medicine physicians. The majority opinion of the five radiology readers served as the final clinical imaging diagnosis.
Performance of algorithm for classifying Alzheimer's disease | ||
Radiology readers | Deep-learning algorithm | |
Sensitivity | 57% | 100% |
Specificity | 91% | 82% |
On the test set, the algorithm yielded 100% sensitivity and 82% specificity for predicting Alzheimer's disease at an early stage -- more than six years before actual diagnosis, on average. The difference in sensitivity between the readers and the algorithm was statistically significant (p < 0.05).
"We did not expect that the model would be able to maintain such high specificity of [more than] 80% [at] 100% sensitivity, but our deep-learning model was able to pick up some subtle correlated features in the brain that human eyes are not able to easily capture," Sohn said. "What surprised us and also did not surprise us is that on the saliency map, which shows which image pixels are more meaningful to the deep-learning algorithm, the algorithm did not focus on a few classical areas involved by Alzheimer's disease, but on the brain as a whole. This goes with the theory that Alzheimer's disease is a diffuse process that involves the brain as a whole."
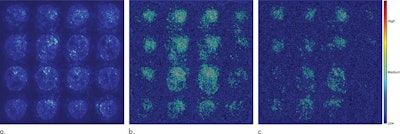
The algorithm now needs to be further validated in a larger, multi-institutional, prospective study, according to Sohn.
He also noted that the deep-learning model still has room for performance improvement in terms of hyperparameter optimization, especially if it's trained with a larger and more diverse clinical dataset. In addition, the researchers are exploring the option of applying the algorithm to other types of PET scans -- such as beta-amyloid plaque and tau protein PET imaging -- with tracers that are more specific to Alzheimer's disease.