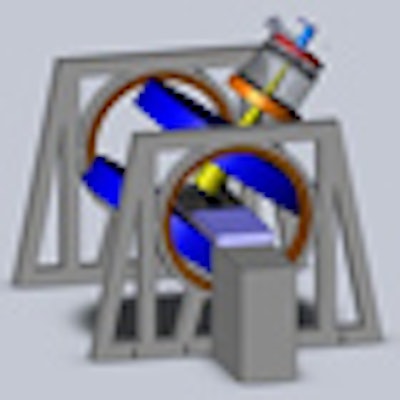
At the recent American Association of Physicists in Medicine (AAPM) annual meeting held in Charlotte, NC, B. Gino Fallone, PhD, provided his annual update of the latest results from the hybrid linear accelerator-MRI system being developed at the Cross Cancer Institute in Edmonton, Alberta. Fallone is director of the University of Alberta Department of Medical Physics.
The hybrid system comprises a 6-MV linac mounted on the open end of a biplanar, low-field MRI magnet, with both the linac and magnet sited on a gantry that rotates around the patient. In this "rotating-biplanar" geometry, the magnetic field vector is fixed with respect to the beam direction. The team at the Cross Cancer Institute has already built and tested a fully functioning head system, and is now constructing and installing a whole-body version using a 0.6-tesla superconducting MRI system.
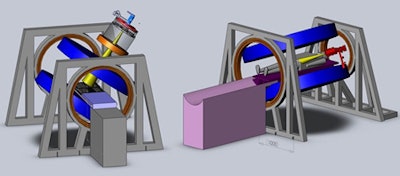
Fallone described the various steps required to perform real-time tumor tracking. The MR system images the tumor during irradiation; the tumor is automatically contoured, its position is determined, and future positions predicted; and then the multileaf collimator leaves are adjusted to account for any motion during the treatment. This process repeats throughout radiation delivery.
Autocontouring
Prior to treatment, the physician defines a region of interest on an MR image of the tumor. During irradiation, an autocontouring algorithm combines this region of interest with information on the range of motion (determined pretreatment over a few breathing cycles) to establish the tumor position.
Fallone and colleagues evaluated their autocontouring algorithm using a lung tumor motion phantom. They scanned the moving phantom using 3-tesla MRI with images obtained at 4 frames/sec, as recommended for lung tracking. Two tumor shapes and four motion patterns were studied, with 600 images recorded for each. The researchers then processed the 3-tesla images to approximate those recorded at 0.2 and 0.5 tesla.
The algorithm successfully contoured the moving tumors, with Dice coefficients (a measure of similarity) of above 0.96 and above 0.93 for the 0.5- and 0.2-tesla images, respectively. The autocontoured centroid positions agreed with the actual positions (provided by an optical encoder on the phantom) to within root-mean-squared errors of 0.92 mm at 0.2 tesla and 0.55 mm at 0.5 tesla.
The researchers also performed an in vivo study, in which a lung cancer patient was scanned with 3-tesla MRI at 4 frames/sec to create 650 MR images. As before, the images were then degraded to simulate low-field images, and the autocontoured shape and its centroid were compared with reference images. In this study, the tumor was contoured with a Dice coefficient of 0.84 for the 0.2-tesla image and 0.86 for the 0.5-tesla image.
Fallone pointed out that the autocontouring process takes less than 5 msec per image, and noted that conventional dynamic lung imaging sequences provide sufficient tumor-tissue contrast-to-noise ratio and temporal resolution for real-time tumor tracking at 0.2 and 0.5 tesla. "Even though these images are more noisy at 0.2 tesla, the software can still pick the image out," he said.
Motion prediction
The process of MR signal acquisition, image reconstruction and processing, and multileaf collimator motion takes approximately 220-280 msec. Thus a means of motion prediction is required to compensate for tumor motion during this system delay. To do this, Fallone's team is applying artificial neural networks, using seven separately trained artificial neural networks to predict tumor positions at 40-msec intervals.
To assess the entire tumor tracking process, the researchers performed an evaluation experiment on the prototype linac-MRI system using a phantom undergoing sinusoidal motion. The phantom was imaged using 0.2-tesla MRI (at 4 frame/sec), the autocontouring algorithm determined the centroid position, and the resulting data were then used to control the motor on a 10-leaf multileaf collimator. The radiation beam was incident upon the phantom for two minutes, with radiographic film placed on the phantom revealing the pattern of irradiation.
When no tracking was applied, the image recorded on the film was, as expected, highly blurred. Tracking without motion prediction improved the image, but some blurring occurred due to the dead time. Adding motion prediction to the tracking resulted in a sharp image with well-defined edges.
The researchers repeated the experiment using a phantom that moved with a modified cosine pattern (which roughly approximates breathing). The 20% to 80% profile of the dose penumbra, a measure that indicates the sharpness of dose deposition, was 6.6 mm for the stationary phantom and 34.1 mm for a moving phantom with no tracking. Tracking without motion prediction led to a 20% to 80% penumbra of 15.8 mm, while tracking with motion prediction improved this further to 8.6 mm.
"The system really requires the seven artificial neural network motion-prediction algorithm to ensure that we know exactly where the target is," Fallone concluded.
ยฉ IOP Publishing Limited. Republished with permission from medicalphysicsweb, a community website covering fundamental research and emerging technologies in medical imaging and radiation therapy.