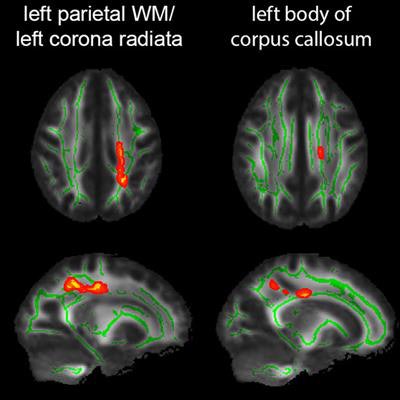
Can the combination of a machine-learning algorithm and diffusion MRI metrics of brain white-matter microstructure serve as a useful method for the early prediction of working-memory impairment? It appears possible, according to recent research from NYU Langone Medical Center in New York City.
In their small proof-of-principle study, the researchers found that two diffusion MRI metrics -- axonal water fraction (AWF) and mean kurtosis (MK) -- had a strong correlation with the performance of healthy adults on a working-memory test. A machine-learning model was then able to utilize those MRI metrics to predict working-memory test results in individuals, with a promising level of accuracy, according to Sohae Chung, PhD, a research scientist.
"Our study elucidates the underlying relationship between white-matter microstructure -- as assessed by multishell diffusion MRI -- and performance on working-memory tasks, and it raises the potential utility of diffusion metrics to predict impairment of working memory," she told AuntMinnie.com.
Chung presented the research in a scientific session at the RSNA 2017 meeting in Chicago.
Essential for brain function
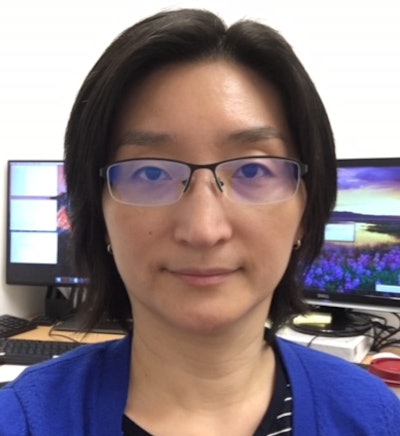
Working memory involves both short-term storage and manipulation of information, and it is essential for higher-order functions such as comprehension, learning, reasoning, and decision-making. Furthermore, deficits in working memory are fundamental problems associated with a wide range of progressive and nonprogressive conditions, including developmental disorders, learning disabilities, traumatic brain injury, stroke, and multiple sclerosis, Chung said.
Very little is known, however, about how working memory relates to the underlying microstructure of brain white matter, she said.
"Elucidating the links between brain microstructure and working memory has generalizeable value across normal and pathologic conditions such as aging, dementia, and abnormalities of attention including attention deficit hyperactivity disorder," Chung said.
Machine learning
As a result, the researchers sought to apply machine-learning techniques to predict working-memory performance in healthy adults by analyzing their white-matter microstructure on multishell diffusion MRI. The project included 15 right-handed and native English-speaking participants, seven of whom were men. The subjects ranged in age from 19 to 45, with an average age of 31. All received a multishell MRI scan on a Skyra 3-tesla MRI scanner (Siemens Healthineers).
A total of 11 diffusion MRI metrics were calculated, including four diffusion-tensor imaging metrics (fractional anisotropy, mean diffusivity, axial diffusivity, and radial diffusivity), three diffusion-kurtosis imaging metrics (mean kurtosis, axial kurtosis, and radial kurtosis), and four compartment-specific white-matter integrity metrics (axonal water fraction, intra-axonal diffusivity, extra-agonal axial diffusivity, and extra-agonal radial diffusivity).
The researchers then used Wechsler Adult Intelligence Scale (WAIS) IV tests to assess working-memory performance. The WAIS-IV Digit Span (DS) test involves asking subjects to perform three tasks: repeating a set of numbers in the order they are presented, repeating a set of numbers in reverse, and repeating numbers in an ascending order. Participants also completed the WAIS-IV Letter-Number Sequencing (LNS) test, which involves receiving a set of numbers and letters and then repeating the numbers first in ascending order, followed by the letters in alphabetical order. The LNS test is the most complex and demanding WAIS-IV working memory subtest, Chung said.
Using tract-based spatial statistics (TBSS) software, the researchers performed skeletonized voxel-wise analysis to determine the areas of significant correlation between the diffusion metrics and the DS/LNS z-scores. Age and gender were used as covariates in the analysis.
Significant correlations
The researchers found significant correlations (p < 0.05) for the AWF and MK results with WAIS-IV LNS test z-scores, indicating that these metrics correlated with working-memory performance. They did not find a significant correlation between any of the diffusion metrics and the WAIS-IV DS test results.
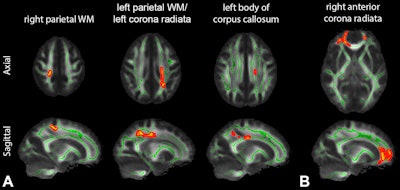
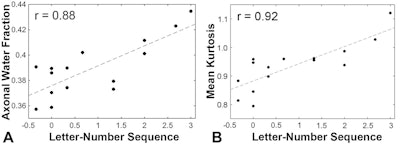
A higher AWF result indicates a higher axon volume, as well as greater myelination, Chung noted. In addition, a higher MK value reflects greater tissue microstructural complexity.
"Our findings support a real potential of diffusion metrics not only to identify white-matter microstructural associations with working memory, but also to begin to parse out the meaning behind such relationships," she said.
Machine learning
After using the TBSS analysis to find correlations between the diffusion metrics and the working-memory test results, the researchers used the average values of the significant image voxels for each diffusion metric as the input features for a machine-learning algorithm. The patient's age was also included in the model, which used gradient-boosted regression trees to predict the LNS score. The model also used a logistic regression classifier to classify the subjects into two categories: those with a predicted DS/LNS z-score of 0.5 or less -- indicating a lower level of working-memory performance -- and those with a predicted score of more than 0.5.
The researchers then tested the algorithm's performance using the leave-one-out and fivefold cross-validation methods. It yielded a prediction root-mean-square error -- a measure of accuracy -- of approximately 83% and a classification accuracy of approximately 73%.
In the next phase of their work, Chung and colleagues plan to explore the use of artificial-intelligence techniques and diffusion MRI metrics on data from the Human Connectome Project (HCP), which offers behavioral data and high-resolution 3-tesla MRI data for 1,200 healthy subjects. After processing the datasets, they will employ deep convolutional neural networks based on the VGG network (VGGNet) architecture, which has successfully been applied to MR image analysis, Chung said.
"We expect that the VGGNet is well-suited for microtextures (such as diffusion parametric maps) since it uses small filters," she said.