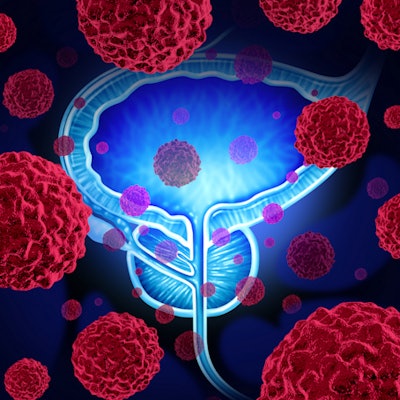
Working with radiomics data on multiparametric MRI (mpMRI) scans, an artificial intelligence (AI) algorithm was able to distinguish between low-risk and high-risk prostate cancer more accurately than the current risk assessment approach used by radiologists, according to research published online February 7 in Scientific Reports.
After testing many different types of machine-learning classifiers, researchers found that the best-performing classifier could, in some aspects, outperform risk assessments produced using Prostate Imaging Reporting and Data System (PI-RADS), version 2. The study came from Icahn School of Medicine at Mount Sinai in New York City and Keck School of Medicine at the University of Southern California (USC).
"By rigorously and systematically combining machine learning with radiomics, our goal is to provide radiologists and clinical personnel with a sound prediction tool that can eventually translate to more effective and personalized patient care," senior author Gaurav Pandey, PhD, said in a statement from Mount Sinai. "The pathway to predicting prostate cancer progression with high accuracy is ever improving, and we believe our objective framework is a much-needed advancement."
Currently, the standard method for assessing prostate cancer risk involves mpMRI for detecting prostate lesions and PI-RADs v2. Radiologists classify lesions seen on mpMRI using the PI-RADS five-point scoring system to predict the likelihood of clinically significant prostate cancer.
However, PI-RADS v2 scoring is subjective and doesn't clearly distinguish between intermediate and malignant cancer levels (scores 3, 4, and 5), according to the researchers. This can lead to variability in interpretations.
As a result, Pandey; first author Bino Varghese, PhD, of USC; and colleagues developed a predictive framework that systematically assessed many types of machine-learning methods using 110 radiomic features. The best classifier -- quadratic kernel-based support vector machine (QSVM) -- was then evaluated on a testing set of 54 prostate cancer patients and compared with PI-RADS v2 classification results.
While PI-RADS v2 classification had a slightly higher overall area under the curve (AUC) than the machine-learning classifier (0.73 versus 0.71), the classifier yielded better overall results when the researchers assessed performance using measures more appropriate for cohorts that are unbalanced -- i.e., there were far more low-risk cases than high-risk ones.
Performance of AI by prostate cancer risk type | ||||
Low-risk prostate cancer patients | High-risk prostate cancer patients | |||
PI-RADS v2 | Machine-learning radiomics classifier | PI-RADS v2 | Machine-learning radiomics classifier | |
F-measure | 0.82 | 0.85 | 0.52 | 0.69 |
Precision | 0.87 | 0.94 | 0.45 | 0.57 |
Recall | 0.78 | 0.78 | 0.61 | 0.86 |
"The goal of incorporating machine learning into radiomics is not to compete with the radiologist, but to rather provide the radiologist and physician team taking care of the patient with objective prediction tools that can aid personalized decision-making regarding individual disease course and treatment outcome," the authors wrote. "The use of such decision-support systems will enhance the quality of the radiologist's work and ... in the long-run aid in the integration of such systems into routine patient care."