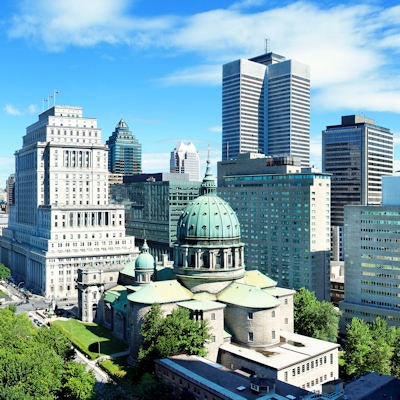
MONTREAL - The number of deep-learning algorithms available for radiology applications is rapidly increasing, and it's time to figure out how to make these tools clinically relevant, according to a presentation Tuesday at the International Society for Magnetic Resonance in Medicine (ISMRM) annual meeting.
Rather than thinking about how an algorithm can solve a particular clinical problem, we need to focus on how this technology can fit into a clinical environment, said Dr. Bradley Erickson, PhD, of the Mayo Clinic in Rochester, MN.
"You're going to see [a] rapid upstroke of clinical applications that are available to us, and I think it's just going to be an exciting new world," Erickson said.
He noted five main categories of clinical applications for deep learning in radiology:
- Regression: Predicting a continuous variable from inputs, such as predicting age from a hand radiograph
- Segmentation: Identifying which pixels are part of a structure of interest, identifying which pixels are abnormal within an identified structure, and labeling each pixel in an image with its type (semantic segmentation)
- Classification: Predicting the nature of a group of pixels, such as determining tumor versus normal, and malignant versus benign
- Generative tasks: Creating new images based on current images
- Workflow and efficiency: Reducing dose and acquisition time
A number of interesting applications exist for regression tasks, including the potential for deep learning to determine how patients are doing based on their images, according to Erickson. For example, a recent paper found that an algorithm was able to predict brain age from a brain MRI.
"We could, for instance, find outliers based on this type of technology when the brain age doesn't match," he said. "This follows from the idea of bone age [assessment]."
A revolution in radiology
Deep learning-performed segmentation will spark a revolution in the practice of radiology, enabling segmentation to be routinely performed, Erickson said. In work at the Mayo Clinic, researchers have explored the use of deep learning for segmentation of body composition, such as separating visceral fat versus subcutaneous fat versus muscle wall thickness.
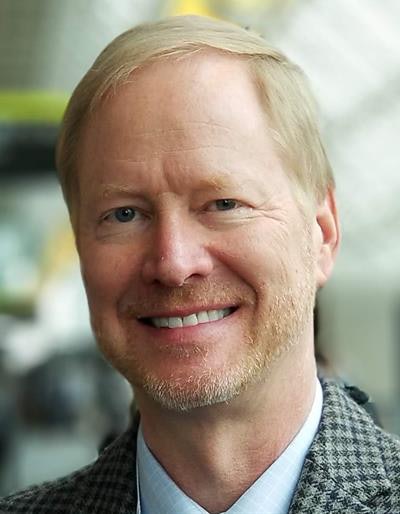
"It's a good measure of how robust a patient is," he said. "So the clinical application that we see right now is for [assessing patient] robustness for surgery. If someone is contemplating a major surgery, these numbers are actually very predictive of how well the patient will do coming through a major surgery. The surgeons are very interested, and this is one of the tools that we are starting to roll out into our clinical practice now."
Segmentation can be useful in a variety of applications, such as in abdominal CT studies. Researchers at the Mayo Clinic have found a deep-learning algorithm can produce Dice scores ranging from 0.96 in the liver and 0.7 for adrenal glands and renal veins. That's approaching a human-level performance, Erickson said.
"I think this is a tool that we are going to see routinely applied in clinical practice because there's so much information that can be gained from this," he noted.
Deep learning can also perform fully automatic segmentation of 132 structures of the brain in only a few minutes and also at a performance nearing the level of humans, according to Erickson. This will be an application that will routinely be utilized in clinical practice and will also yield important new biomarkers, he said.
Other segmentation tools
Another segmentation tool that's being rolled out into clinical practice calculates total kidney volume from abdominal MRI in patients with polycystic kidney disease, according to Erickson.
"The great thing about deep-learning technology is that it's amazingly robust; even in cases that are so markedly abnormal, it gets it right," he explained. "I think that's one reason that we're going to see much more rapid adoption of deep-learning techniques than we saw for traditional machine-learning methods."
Deep-learning algorithms can also perform tumor segmentation, as well as automated contouring of primary tumor volume on MRI for nasopharyngeal carcinoma.
In the near future, deep learning will routinely segment and quantify medical images, Erickson said.
"And I think [it's] likely that the vendor manufacturers will start to implement that into the scanner themselves," he added. "After all, they know exactly how that image was acquired. They know the parameters, they know how they happen to shape the pulse sequences, which is going to impact contrast in a more subtle way. That's probably three to five years out, but they have the GPU technology already in the scanners. It's just sitting there waiting to be used."
This will lead to a revolution in imaging-based biomarkers, Erickson continued. In addition to volumes and ratios of volumes as biomarkers of disease, there's also a lot more information in the densities, textures, and other properties of organs that are present in the images and could be easily and reliably measured, according to Erickson.
Classification
Deep learning can also be performed for classification tasks, such as identifying the presence of a disease and determining what the disease is. Additionally, it can assess if the image represents progression or response to treatment and can assess molecular/genomic properties, Erickson said.
In musculoskeletal MRI, for example, a deep-learning algorithm called MRNet from a Stanford University group was recently found to improve the performance of radiologists in diagnosing knee injuries on MRI.
"It's not so much man versus machine, but it's man versus man plus machine," he said.
A team in Erickson's lab at the Mayo Clinic also has found that a deep-learning algorithm could yield accuracy ranging from 87% to 95% for four key molecular markers of glioma. "Image-omics" is better than tissue genomics, he noted.
"Tissue-based 'omics' doesn't reveal other parts of the tumor; it's only telling you about the piece of tissue that you submit to the test," he explained. "It doesn't tell you about the phenotype, it doesn't tell you about how the genes are expressed in that particular tumor, and it doesn't tell you about gene-gene interactions. We know that these things are way more complex than one pathway. ... And also we know that some people do better than other people; there's something about [hosts] and the way they fight the tumor that's different. And imaging can reflect all of that, whereas tissue genomics just won't."
That's why radiologists need to be at the core of the fight for precision medicine, Erickson said.
"I think we can add as much information as the pathologist when we study how a patient is going to respond to a given therapy," he noted.
Improving images
Deep-learning algorithms have been developed for improving images, including speeding up acquisition time and outperforming traditional noise reduction techniques in image reconstruction. In particular, generative adversarial networks (GANs) are going to have a huge impact in radiology, according to Erickson.
In fact, the potential of GANs has led Erickson to disagree with the notion that radiology is at the top of the hype cycle for deep learning.
"This GAN technology means that we're at the early part because we're just starting to apply that technology now," he said. "And quite frankly, when I looked through all of the technologies that were present in posters here [at ISMRM 2019] ... there are so many papers that show this rapid advance that is occurring with this generative type of technology for improving the quality of images, for improving the information content, the nature of the images, being able to produce FLAIRs [fluid-attenuated inversion-recovery images] from T1s and T2s, all this sort of thing. We're early on the learning curve for what deep learning can do to clinical practice."
Erickson emphasized that the palette of artificial intelligence (AI) algorithms cleared by the U.S. Food and Drug Administration (FDA) for clinical use has rapidly increased over the last few years, which will, in turn, lead to a swift increase in the number of clinical tools being used in practice.
"I think the possibilities for how this can impact clinical practice really are limited [only] by our imagination," he stated.
Cautionary note
Erickson cautioned, however, that the present state of workflow for AI algorithms in radiology poses some challenges. Many tools use point-to-point connections, such as querying the scanner or PACS for specific classes of exams that it can work on, he said.
Some platforms collect exams and provide an environment for execution of algorithms, but just like in the days before the DICOM Standard, most users often select one vendor platform, Erickson explained.
"As the AI tool palette expands, you're not going to want to be locked into one vendor," he said.
To prevent this, Erickson and other luminaries in the imaging informatics community have begun advancing the concept of vendor-neutral orchestration of analytics and tools.
"We need to have a vendor-neutral orchestration of these AI tools, and I think it needs to be vendor-neutral so that you can always pick best-of-breed and figure out how to connect it in," he said.