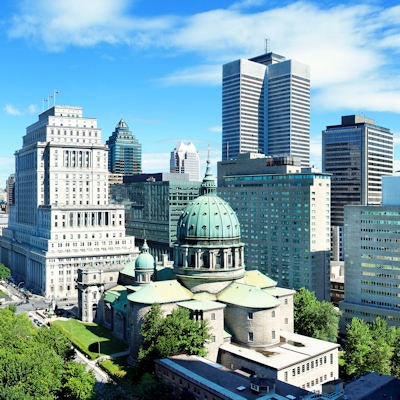
MONTREAL - An artificial intelligence (AI) algorithm can produce high-quality synthetic contrast-enhanced brain MR images, potentially helping to avoid usage of gadolinium-based contrast agents, according to a presentation Thursday at the International Society for Magnetic Resonance in Medicine (ISMRM) annual meeting.
After analyzing a series of noncontrast-enhanced images, an attention generative adversarial network (GAN) can synthesize postcontrast T1-weighted images that greatly resemble the patients' actual contrast-enhanced images, according to co-author and presenter Kevin Chen, PhD, of Stanford University in Palo Alto, CA.
"In addition, the model can successfully predict some enhancing lesions that are not shown in the precontrast images," he said. "With further validation, this approach could have great clinical value, especially for patients who cannot receive contrast injection."
Chen presented the research on behalf of first author Jiang Liu of Tsinghua University in Beijing. The team also included researchers from Grenoble Institute of Neurosciences in France and AI software developer Subtle Medical.
Although gadolinium-based contrast agents (GBCAs) have been used in clinical practice to provide better visibility of pathology and delineation of lesions on MRI, these agents do have several drawbacks, Chen said. For example, some patients, particularly those with severe kidney disease, can't receive GBCAs. In addition, the medical community has become increasingly concerned over the safety of these agents due to linkage with the development of nephrogenic systemic fibrosis and the deposition of gadolinium within the brain and body. These agents also increase the cost of MRI.
In hopes of acquiring the same enhancing information without actually injecting a contrast agent, the researchers sought to apply deep learning to synthesize gadolinium-enhanced T1-weighted images from precontrast images.
"If so, we can potentially avoid using the contrast agents to prevent the side effects [of GBCAs] and reduce cost," Chen explained.
The researchers retrospectively gathered a dataset of 404 adult clinical patients being evaluated for suspected or known enhancing brain abnormalities. This cohort encompassed a wide range of indications, pathologies, ages, and genders, according to Chen. Of these 404 patients, 323 (80%) were randomly chosen for training the model, with the remainder set aside for testing.
All patients were scanned on a 3-tesla MR scanner with an eight-channel head coil (GE Healthcare). The precontrast imaging protocol included acquisition of 3D inversion recovery (IR)-prepped fast spoiled GRASS (FSPGR) T1-weighted images, T2-weighted images, T2*-weighted images, diffusion-weighted images (DWI) with 2b values (0 to 1000), and 3D arterial spin labeling images. Next, 3D IR-prepped FSPGR T1-weighted images were acquired after the patients received 0.1 mmol/kg of gadobenate dimeglumine contrast.
After data preprocessing was performed, the images were used to train the attention GAN, which utilizes convolutional neural networks (CNNs). The GAN received five slices each of the acquired 2.5D precontrast images. Two "blocks" of the U-Net CNN then worked together to serve as the "generator" in the GAN, learning how to synthesize postcontrast images from the inputted precontrast images. A six-layer CNN acted as the GAN "discriminator," which was trained to distinguish the synthetic images from the acquired ground truth post-contrast T1-weighted images, according to Chen.
"This forces the generator to produce high-quality images," Chen said.
Using two similarity metrics, the researchers then evaluated the GAN's performance by comparing the synthetic contrast-enhanced T1-weighted images produced on the testing dataset with the ground truth gadolinium-enhanced T1-weighted images. They found that the synthetic images were highly similar to the actual contrast-enhanced images.
Similarity to actual contrast-enhanced images | ||
Precontrast T1-weighted images | Synthetic contrast-enhanced T1-weighted images | |
Structural similarity index | 0.836 ยฑ 0.055 | 0.939 ยฑ 0.031 |
Peak signal-to-noise ratio | 19.6 ยฑ 6.5 | 27.5 ยฑ 5.6 |
Chen noted, however, that the model still fails to predict some contrast uptakes, a shortcoming that may be caused by the lack of similar cases in the training set.
"In the future, we will need to include more cases with enhancing lesions in the training set to improve the performance," he said. "We are also thinking about using multitasking techniques, for example, combining lesion detection with contrast synthesis."