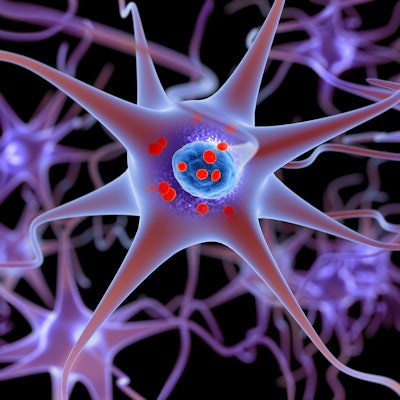
An artificial intelligence (AI) algorithm can distinguish between parkinsonian syndromes on diffusion-weighted MRI (DWI-MRI) exams at an accuracy level comparable to that of current gold-standard methods, according to research recently published online in Lancet Digital Health.
After developing machine-learning models using DWI-MRI exams from over 1,000 subjects, a multinational team of researchers led by Derek Archer, PhD, of the University of Florida at Gainesville, found the best-performing algorithm was highly accurate for distinguishing between Parkinson's disease and atypical parkinsonism -- which is characterized by multiple-system atrophy and progressive supranuclear palsy.
"The diffusion-weighted MRI method does not involve radioactive tracers, is completely automated, and can be collected in less than 12 [minutes] across 3-tesla scanners worldwide," the authors wrote (Lancet Digit Health, August 27, 2019). "The use of this test could positively affect the clinical care of patients with Parkinson's disease and parkinsonism, and reduce the number of misdiagnosed cases in clinical trials."
As they often share motor and nonmotor symptoms, Parkinson's disease and atypical parkinsonism can be challenging to differentiate in a clinical setting, according to the researchers.
"Development of a fully automated, [generalizable] procedure that differentiates parkinsonian syndromes by use of diffusion measurements from pathologically relevant regions is a crucial need for the field," the authors wrote.
As a result, the researchers set out to develop an automated method that can differentiate parkinsonian syndromes on DWI-MRI using data from 1,002 individuals contributed by 17 MRI centers around the world. Of these, 511 (51%) had Parkinson's disease, 278 (28%) were healthy controls, 129 (13%) had progressive supranuclear palsy, and 84 (8%) had multiple system atrophy. Training and validation were performed on 80% of the data with the remaining 20% set aside for testing.
To train machine-learning algorithms, they used free-water and free-water-corrected fractional anisotropy measurements calculated on DWI-MRI across 60 different template regions, including 43 white-matter tracts and 17 regions in the basal ganglia, cerebellum, and cortex. They created three different support-vector machine (SVM)-based machine-learning models: one based on DWI-MRI only, one based only on the Movement Disorders Society Unified Parkinson's Disease Rating Scale part III (MDS-UPDRS III), and one based on both.
The combined model yielded the highest accuracy.
Machine-learning algorithm for distinguishing between parkinsonian syndromes | |
Area under the curve | |
Parkinson's disease vs. atypical parkinsonism | 0.962 |
Multiple system atrophy vs. progressive supranuclear palsy | 0.897 |
In a subset of five cases with postmortem neuropathological examinations, the DWI-MRI diagnosis matched the pathological diagnosis, according to the researchers. They also noted the 10 regions that had the highest relative importance to the model were those previously shown to be pathologically involved in Parkinson's disease, multiple system atrophy, and progressive supranuclear palsy.
"Our results are relevant in the clinical setting because they indicate that diffusion-weighted MRI might provide a biomarker for physicians to use in considering a patient to have atypical parkinsonism or Parkinson's disease and in distinguishing between multiple system atrophy from progressive supranuclear palsy," they wrote. "The outcome of this study suggests that the imaging and machine-learning model might function well using data from new sites."