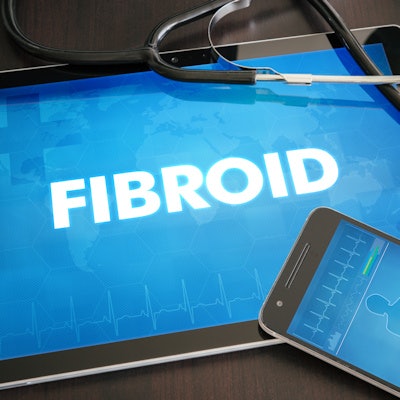
An artificial intelligence (AI) algorithm's analysis of MRI exams performed prior to uterine fibroid embolization (UFE) can be more accurate than radiologists in predicting whether the procedure will be successful, according to research presented at the recent RSNA 2019 meeting in Chicago.
A multinational team of researchers led by presenter Dr. Yong-Heng Luo, PhD, from the Second Xiangya Hospital of Central South University in China reported that their deep-learning algorithm bettered the average predictions of four radiologists in predicting UFE outcomes based on analysis of clinical information and pretreatment MRI exams.
UFE is an interventional radiology procedure that relieves symptoms from uterine fibroids, but there currently isn't an effective modality to predict which patients will respond best to these procedures, Luo said. MRI is commonly performed, however, before and after UFE.
As a result, the researchers wanted to develop and validate a deep-learning model to predict clinical outcomes of UFE based on preprocedure MRI scans. They trained and tested a residual convolutional neural network (ResNet) to predict fibroid volume reduction and clinical outcome using a retrospective cohort of 409 patients with 727 fibroids. All patients included in the study had received preoperative and postoperative MRI.
Using the 3D Slicer software, an abdominal radiologist manually segmented the fibroids for each patient on T2-weighted and postcontrast T1-weighted (T1C) sequences of the preprocedure and postprocedure MRI scans. The reduction in fibroid size was calculated as the percentage of fibroid volume change after uterine fibroid embolization, according to the researchers.
Of the 727 fibroids, 509 (70%) served as the training set and 146 (20%) were used for the validation set. The remaining 72 (10%) cases were set aside as the test set.
The deep-learning algorithms were trained using both imaging and clinical variables, with separate models trained on the T1C images, the T2-weighted images, and the clinical variables. An ensemble model was also created based on the output probabilities generated by each of the individual models.
Four radiologists also reviewed the clinical information of the patients and the T1-weighted, T1C, and T2-weighted images. Blinded to the UFE outcomes, they then independently predicted whether the embolization procedure would be a success or a failure.
Performance of AI for predicting UFE outcomes | |||||
Average of radiologists | AI model trained using clinical data | AI model trained using T1C images | AI model trained using T2-weighted images | Ensemble AI model | |
Sensitivity | 85.2% | 68.6% | 93.2% | 93.2% | 96.6% |
Specificity | 13.5% | 18.7% | 46.2% | 23.1% | 30.8% |
Accuracy | 72.2% | 81.9% | 84.7% | 80.6% | 84.7% |
F1 score | 0.834 | 0.901 | 0.901 | 0.909 | 0.887 |
Area under the curve | N/A | 0.865 | 0.901 | 0.88 | 0.85 |
"[All AI] models achieved higher accuracy in predicting outcome when compared with the radiologists," Luo said. "The most important model is the T1C model."
The researchers acknowledged a number of limitations to their study, including its reliance on data from a single institution and the lack of an advanced MR sequence.