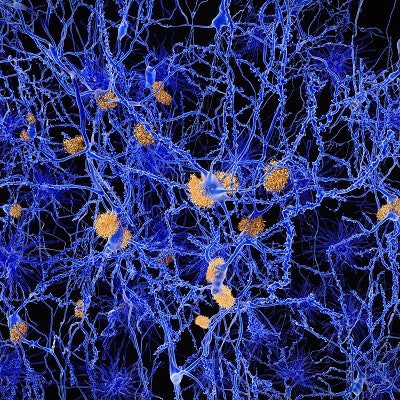
Radiomic features in the hippocampus on MRI scans could serve as biomarkers for predicting if patients will progress from mild cognitive impairment (MCI) to Alzheimer's disease, according to research published online April 3 in Science Bulletin.
A team of researchers led by Kun Zhao of the Chinese Academy of Sciences in Beijing trained a machine-learning algorithm to use these radiomic features to distinguish between Alzheimer's disease and control patients at an accuracy level of up to 88%.
What's more, these hippocampal radiomics features were also associated with clinical features in patients with mild cognitive impairment and demonstrated a consistently altered pattern that correlated with changes in a patient's cognitive ability over a five-year period, according to the researchers.
"These comprehensive results suggest that hippocampal radiomic features can serve as robust biomarkers for clinical application in [patients with Alzheimer's disease or mild cognitive impairment] and further provide evidence for predicting whether an MCI subject would convert to [Alzheimer's disease] based on the radiomics of the hippocampus," they wrote.
Although morphological change in the hippocampus is known as one of the main hallmarks of Alzheimer's disease, it's currently unclear if hippocampal radiomic features could reliably enable prediction of progression from mild cognitive impairment to Alzheimer's disease, according to the researchers.
Using 715 subjects from six sites and an additional independent dataset, they sought to determine if hippocampal radiomic features could be utilized as MRI biomarkers of Alzheimer's disease. The researchers also wanted to test their hypothesis that these radiomic features have a solid neurological basis and therefore demonstrate initial promise as potential markers of Alzheimer's disease traits or prodromal disease states.
The 715 subjects in the study included 261 patients with Alzheimer's disease, 223 patients with mild cognitive impairment, and 231 controls. The authors created a support vector machine algorithm to classify patients based on three types of radiomic features of the hippocampus that were extracted from the MRI exams -- intensity-based, shape-based, and texture-based.
They tested their method with two independent cross-validation methods: using the in-house dataset for training and 1,228 subjects from the Alzheimer's Disease Neuroimaging Initiative (ADNI) dataset for testing, and vice versa.
Performance of machine-learning algorithm for classifying Alzheimer's disease | |||
In-house intersite cross-validation | Trained on in-house data and tested on ADNI | Trained on ADNI data and tested on in-house data | |
Accuracy | 88% | 79% | 84% |
Area under the curve | 0.95 | 0.89 | 0.92 |
The researchers found that the radiomic features appeared to represent a robust, reproducible, and generalizable imaging signature of Alzheimer's disease.
"Moreover, the enrichment analyses of the association between radiomic features and the genotype, Tau, Aβ and longitudinal cognitive variances highlight the solid neurobiological substrates underlying the progression of [Alzheimer's disease]," they wrote. "This is of great significance for the early clinical diagnosis or prognostic follow-up in [Alzheimer's disease]."
The researchers noted that "the use of radiomic features of the hippocampus alone are not enough to fully describe [Alzheimer's disease] progression; combining markers from other brain regions will help identify robust and reproducible biomarkers for clinical application."