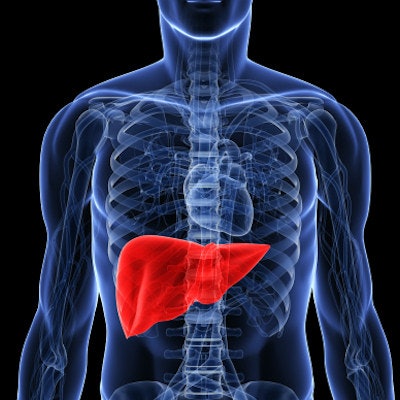
An artificial intelligence (AI) algorithm improved MRI's performance when it comes to tracking changes in liver lesions after therapy has started, according to a study published July 25 in the Journal of the American College of Radiology.
The findings suggest that AI could streamline radiologist workflow and free them up to devote their time to more complex tasks, wrote a team led by Dr. Alexander Goehler, PhD, of Beth-Israel Deaconess Medical Center and Harvard Medical School in Boston.
"With recent advances in neural networks for medical imaging, the possibility to automate segmentation tasks offers the promise of helping clinical radiologists by completing tedious tasks more quickly and comprehensively and thus allowing them more time to focus on higher-level interpretive and cognitive tasks," the team wrote.
Tumor response to therapy is often evaluated by tracking changes in liver lesion size using MRI, but these exams can be time-consuming, the team noted. Goehler and colleagues investigated whether AI for detecting liver metastases on MRI could effectively assess changes in tumor size on ensuing exams and therefore evaluate patients' therapy response.
The study included 64 patients with neuroendocrine tumors who had at least two consecutive liver MRI exams with gadoxetic acid. The researchers used a 3D neural network to first identify and segment the liver and then identify lesions within the organ; they analyzed the algorithm's performance compared with human readers in part by using the Dice similarity coefficient (for which scores closer to 1 equal higher similarity between two samples). Data used to train the algorithm included 691 liver lesions (i.e., 188 liver segments with lesions); of these, 160 were true-positive for malignancy and 141 were true-negative.
The AI algorithm agreed with two reading radiologists' manual assessment of liver lesion change on MRI exams 91% of the time, with high sensitivity, specificity, and accuracy rates for classifying liver segments as malignant or benign. The mean Dice coefficient scores for individual lesions (comparing the algorithm's and human readers' performances) ranged from 0.73 to 0.81.
AI algorithm's performance in identifying diseased versus healthy tissue in liver segments | |
Performance measure | AI algorithm |
Accuracy | 88% |
Sensitivity | 85% |
Specificity | 92% |
The algorithm's potential isn't just in streamlining workflow but also providing a depth of data that can be difficult to tease out in the midst of a busy clinical day, the authors noted.
"The true potential of the algorithm goes beyond its faster performance compared with a human interpreter and includes the potential to perform an entire volumetric tumor burden assessment," they concluded. "Although these have not been feasible to perform manually outside of small exploratory research trials, artificial intelligence-based detectors will likely be able to perform such tasks as part of clinical routines."