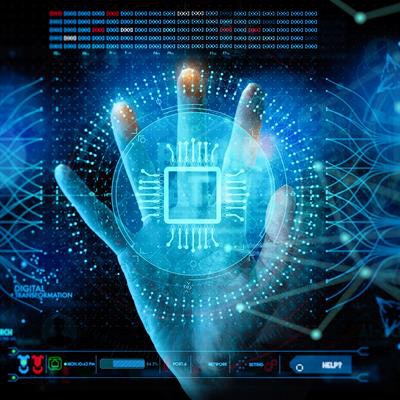
Federated learning -- an approach to training deep-learning models that incorporates data from other sites without sharing the actual data -- can protect patient privacy and enable more-generalizable radiology artificial intelligence (AI) algorithms, according to research published online July 28 in Scientific Reports.
A team of researchers led by senior author Spyridon Bakas, PhD, of the University of Pennsylvania, and first author Micah Sheller of Intel utilized federated learning to train an AI algorithm to detect tumors on brain MRI scans using data from 10 healthcare institutions. A consensus algorithm aggregated from each site's individual results achieved 99% of the performance of a model that was trained once using the same data that had been pooled together in a repository. It also performed better than other data-sharing approaches that are likewise geared toward protecting patient privacy.
"Clinical adoption of federated learning is expected to lead to models trained on datasets of unprecedented size, hence have a catalytic impact towards precision/personalized medicine," wrote the authors, which included researchers from the University of Texas M.D. Anderson Cancer Center in Houston, Washington University in St. Louis, and the University of Pittsburgh.
Creating a consensus model
The researchers initially pretrained a convolutional neural network using a multi-institutional dataset of over 2,600 brain MRI scans from 660 patients included in the International Brain Tumor Segmentation (BraTS) challenge. Next, 10 hospitals trained versions of this AI model with their own patient data. Via a federated learning method, these updated models were then aggregated to create a consensus model.
Using validation datasets that had not been used for training, the researchers then compared the performance of the consensus model with that achieved by the models trained at each institution, as well as an algorithm trained via collaborative data sharing, a method in which participating sites would all share their patient data to a centralized data repository. They also assessed other collaborative approaches that protect patient privacy, such as institutional incremental learning -- training the model at one institution and then passing it on to the next for subsequent training -- and cyclic institutional incremental learning, which adds repeated cycles of training through the various sites.
Performance of AI models by training method in comparison with ground truth | |||||
Average for single-institutional models | Average for institutional incremental learning | Best average local result for cyclic institutional incremental learning | Average for collaborative data sharing | Average for federated learning | |
Dice similarity coefficient (DSC) | 0.749 | 0.810 | 0.840 | 0.850 | 0.846 |
Despite slightly lower performance, federated learning has the advantage of providing raw data confidentiality, according to the researchers. By incorporating additional current technologies to alleviate additional privacy concerns, federated learning will enable large-scale collaborative training of algorithms with unprecedented amounts and diversity of data, they said.
"Such collaborations are likely to result in a significant jump in the state-of-the-art performance for these models," the authors wrote.
Project expansion
The results of the study led to an expanded collaboration between Penn Medicine, Intel, and 30 partner institutions across nine countries aimed at using the federal learning approach to train a consensus AI model on brain tumor data. The U.S. National Cancer Institute (NCI) has also backed the initiative with a $1.2 million grant. The goal is to create an open-source tool that can be used by any clinician at any hospital, according to the researchers.
The study and the larger federated-learning project open up possibilities for expanded utilization of AI, including radiomics, according to co-author Dr. Rivka Colen of the University of Pittsburgh School of Medicine.
"Radiomics is to radiology what genomics was to pathology," she said in a statement. "AI will revolutionize this field, because, right now, as a radiologist, most of what we do is descriptive. With deep learning, we're able to extract information that is hidden in this layer of digitized images."