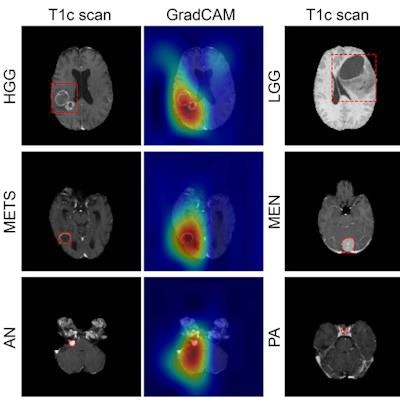
A deep-learning model was able to classify brain tumors as one of six common types on a single 3D MRI scan, according to a study published August 11 in Radiology: Artificial Intelligence.
The findings could translate to improved workflow and better brain tumor diagnosis, wrote a team led by doctoral student Satrajit Chakrabarty of Washington University School of Medicine in St. Louis, MO.
"This network is the first step toward developing an artificial intelligence-augmented radiology workflow that can support image interpretation by providing quantitative information and statistics," he said in a statement released by the RSNA.
There are six common brain tumor types: high-grade glioma, low-grade glioma, brain metastases, meningioma, pituitary adenoma, and acoustic neuroma, the authors noted. Researchers have been exploring the use of machine and deep learning to classify tumors but haven't necessarily included all six of these -- or tried to distinguish healthy scans from those that show tumors.
In the current study, the authors sought to address this knowledge gap by building and testing a convolutional neural network using data from 2,105 3D brain MRI scans. Of these, 1,396 were used to train the network to distinguish between healthy scans and those with tumors, while 361 were used for testing an internal set of images (from Washington University) and 348 for testing an external set (from Brain Tumor Segmentation [BraTS] 2018, BraTS 2019, and LGG 1p19q from the Cancer Imaging Archive).
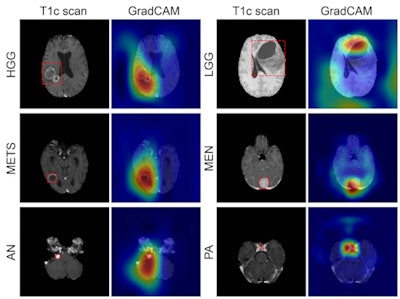
The model showed high performance across seven imaging classes (the six tumor type groups and one healthy group) and a variety of measures in the Washington University test set. The external test data had only two tumor types -- high- and low-grade glioma -- and showed an accuracy of 92%.
Performance of deep-learning model for classifying brain tumors | ||
Measure | Internal test data | External test data |
Accuracy | 93.4% | 92% |
Area under the receiver operating curve | 0.98-1.00 | 0.97-0.98 |
Negative predictive value | 98%-100% | 72%-99% |
Positive predictive value | 85%-100% | 73%-99% |
Sensitivity | 81%-100% | 91%-97% |
Specificity | 97%-100% | 91%-97% |
The model streamlines radiology workflow by eliminating the need for manual brain tumor segmentation before classification, according to the team. The group hopes to use it with additional tumor types in future research.
"These results suggest that deep learning is a promising approach for automated classification and evaluation of brain tumors," Chakrabarty said in the RSNA statement. "The model achieved high accuracy on a heterogeneous dataset and showed excellent generalization capabilities on unseen testing data."