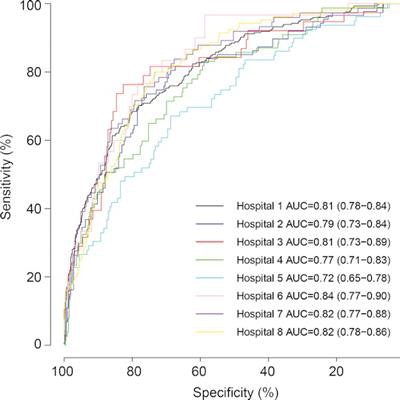
A deep-learning model dismissed nearly 40% of breast MRI scans without lesions while not missing any cancers in women with dense breasts, offering hope as a triage tool, according to research published October 5 in Radiology.
A team led by Erik Verburg of Utrecht University in the Netherlands said the model successfully differentiated between women with and without breast lesions in a study of nearly 4,600 individuals. The authors added that to their knowledge, this study is the first to investigate image-based triaging with use of multicenter screening data of women with extremely dense breasts at average risk.
"We did not know what to expect because no studies had done anything like this before as far as we know," said co-author Dr. Kenneth Gilhuijs, PhD, from the university to AuntMinnie.com. "Especially in a screening population of women with very dense breasts, unclear enhancement on MRI occurs relatively often, posing major challenges."
MRI screening is a preferred method for imaging women with dense breasts thanks to its higher sensitivity. In the Netherlands, about 8% of women participating in screening programs have extremely dense breasts. Nearly 82,000 women are eligible for MRI biennial breast screening in the Netherlands alone.
The researchers said routine use of MRI to screen women with extremely dense breasts on a population scale would be challenging.
"Most MRI examinations show normal anatomic and physiologic variation that may not require radiologic review," they said. "Thus, ways to triage these normal MRI examinations to reduce radiologist workload are needed."
The team wanted to find out how feasible automated triaging is by using their deep-learning model that analyzed screening breast MRI scans. The group wanted to see if artificial intelligence could reduce workload and prioritize the work of breast MRI radiologists by dismissing the largest number of examinations without lesions while still identifying all scans with malignant disease.
The researchers tested the model on imaging data pulled from 4,581 MRI examinations of extremely dense breasts from 4,581 women with an average age of 54.3 years. The women were part of the Dense Tissue and Early Breast Neoplasm Screening (DENSE) trial between 2011 and 2016.
Of the 9,162 breasts examined, 838 had at least one lesion that ranged from BI-RADS category 2 through 5 (77 being malignant) and 8,324 had no lesions.
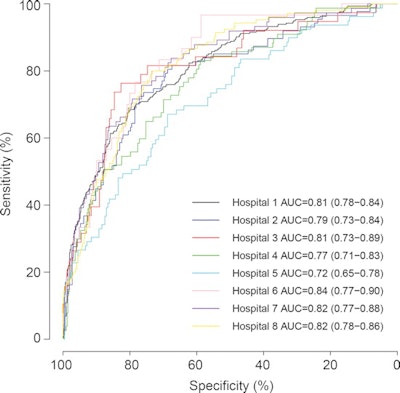
At 100% sensitivity for malignant lesions, the deep-learning model considered 90.7% of the MRI examinations with lesions to be suspicious and triaged them to radiologic review. The model meanwhile dismissed 39.7% of the MRI examinations without lesions.
The model also had an average area under the receiver operating characteristic curve of 0.83 in differentiating between normal breast MRI examinations and MRI examinations with lesions.
The team wrote that the study's findings are significant and will trigger follow-up studies to confirm the safety of AI and its noninferiority to well-trained breast MRI radiologists, as well as help merge artificial intelligence into the clinical workflow in the "foreseeable" near future.
"Given the high volume of examinations that nationwide screening of women with dense breasts would bring, safely dismissing nearly half the number of normal scans would already amount to a relatively large absolute reduction in workload," Gilhuijs told AuntMinnie.com.
The team is working on validation studies in follow-up screening rounds, further expanding the training database of the model.
"We are also looking into other screening indications for breast MRI," Gilhuijs added.