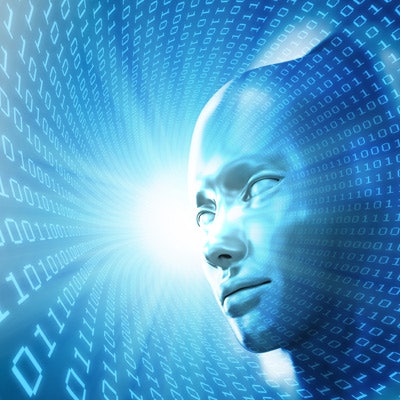
A deep learning-based risk assessment model can perform better than traditional risk models for guiding decisions on supplemental breast MRI screening, according to a talk at the Society of Breast Imaging/American College of Radiology symposium.
In a retrospective study involving over 2,000 women, a team of researchers from Massachusetts General Hospital led by presenter Dr. Kimeya Ghaderi found that risk assessment performed on a deep learning-based model would have led to fewer patients categorized as having intermediate five-year risk or long-term high-risk -- without sacrificing on positive predictive values, sensitivity, or specificity.
"A deep learning risk-assessment model can support more effective supplemental breast MRI screening compared to traditional risk assessment models, as measured by the cancer detection rates and the positive predictive values," Ghaderi said.
Screening breast MRI is currently recommended in high-risk patients. However, multiple studies have shown imprecise utilization; screening breast MRI is often overused in patients at average risk for breast cancer and underutilized in patients at high risk, according to Ghaderi.
Risk assessment has traditionally been performed using models such as the Tyrer-Cuzick (TC8) model and the U.S. National Cancer Institute (NCI) Breast Cancer Risk Assessment Tool/Gail model. Both of these methods are largely dependent on family history, she said.
"New and emerging deep-learning models around breast cancer risk stratification have been shown to outperform that of traditional risk assessment models," she said. "Therefore, deep-learning models may better identify those at high risk of breast cancer and therefore, most likely to benefit from supplemental MRI screening."
In their study, the researchers sought to assess the impact of a deep-learning model to help support more effective MRI screening. They retrospectively gathered consecutive screening breast MRI exams performed at the four sites of their tertiary academic institution between September 2017 to September 2020.
All women included in the study had received a screening mammogram in the previous 24 months, were ≥ 40 years old, had a one-year follow-up, and had at least one valid risk score. Those missing a specific indication for the breast MRI scan were excluded.
The researchers set the deep-learning risk score thresholds to match the percentage of patients in the mammogram population labeled by the TC8 model as intermediate five-year risk ≥ 1.67% and lifetime high risk ≥ 20%. This resulted in a deep-learning score threshold of 2.3 for five-year intermediate risk and 6.6 for a lifetime high risk.
The final analysis dataset in the study included 4,016 screening breast MRI exams from 2,231 women. The patients had an average age of 54 and 91.8% identified as Caucasian.
Almost 67% of patients had dense or heterogeneous breast tissue. Furthermore, 71% had a personal history of breast cancer and 77.2% had a family history of breast cancer.
Of those undergoing screening breast MRI, 78.1% of patients by the TC8 model were considered to have intermediate five-year risk, compared with 77.1% of patients by the NCI model and 53.8% of patients by the deep-learning model. In addition, 51.7% of patients were considered to have a lifetime high-risk (≥ 20%) for breast cancer by the TC8 model, compared with 36.2% on the NCI model and 14.1% on the deep-learning model.
The cancer detection rate was higher among the women identified by the deep-learning model as either lifetime high-risk or an intermediate five-year risk.
Performance of deep-learning models in intermediate/high-risk patients receiving screening breast MRI | ||||||
Tyrer-Cuzick: Intermediate 5-year risk | NCI model: Intermediate 5-year risk | Deep learning: Intermediate 5-year risk | Tyrer-Cuzick: Lifetime risk ≥ 20% | NCI model: Lifetime risk ≥ 20% | Deep learning: Lifetime risk ≥ 20% | |
Cancer detection rate | 6.8% | 5.3% | 16.7% | 6% | 6.8% | 19.4% |
In other results, the deep-learning model also yielded higher PPV1, PPV2, and PPV3 compared with the TC8 and NCI models (p ≥ 0.05) for both intermediate five-year risk and high long-term risk. There were also no significant differences in abnormal interpretation rate, sensitivity, or specificity compared with the traditional models.
Ghaderi noted that the deep-learning risk score has the advantage of being automatically generated at the time of the screening mammogram. It also doesn't have the same limitations as traditional risk-assessment models, including exclusion of patients with personal history of breast cancer, patients with no genetic mutations, and patients with unknown family history.
"Furthermore, the deep-learning model also does not need any additional resources to aid in data acquisition and performs without any inherent racial bias," she said. "In the future, we look forward to seeing the impact of our research on patients and providers and providing a more appropriate guidance of high-risk patients for supplemental breast screening MRI."