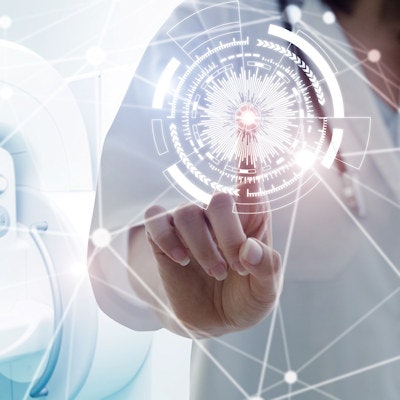
Breast density continues to be a challenge for breast radiologists, but recent research sheds new light on how breast imagers can refine cancer risk estimates for women with dense breast tissue.
In presentations at ECR 2022, Dr. Nicholas Payne from the University of Cambridge in the U.K. and Dr. Riccardo Samperna from Radboud University Medical Center in the Netherlands discussed their respective teams' work on this topic, which included creating risk scores and bridging gaps in automatic breast density estimation.
"It's a question at the moment on whether we should change or alter the way in which we screen women who have dense breast tissue, as dense tissue increases the risk for breast cancer and masks more lesions on mammograms," Payne said.
Breast density is a known risk factor for boosting a woman's chances of developing breast cancer, with conventional mammography struggling to identify breast cancer in highly dense breasts. Digital breast tomosynthesis (DBT) and supplemental screening methods such as MRI and ultrasound have been proposed to help in this area. Indeed, the European Society of Breast Imaging (EUSOBI) recommends that women with extremely dense breasts undergo supplemental screening, preferably with MRI.
But just how at risk are women with varying breast densities?
In his ECR presentation, Payne talked about his team's study, which looked at data from the multisite Breast Screening: Risk Adaptive Imaging for Density (BRAID) trial. The trial includes women with dense breasts (BI-RADS C or D) and who attend routine breast screening.
Women in the trial completed an online questionnaire covering physiology, lifestyle, medical history, and family history. The team collected data from a total of 3,706 women between the ages of 50 and 70.
From there, researchers fed the data into an algorithm the university created, called the Breast and Ovarian Analysis of Disease Incidence and Carrier Estimation Algorithm (BOADICEA). This generated a score representing the risk of breast cancer over the next five years.
They found that the cancer risk score generated by BOADICEA based on multiple variables changed risk estimates as opposed to using a single variable alone.
Breast cancer risk estimate based on BOADICEA risk score vs. individual variables | ||||
Risk level | Thresholds | Age | Age and density | BOADICEA risk score based on all available data |
Low risk | < 1% | 0% | 0% | 11.9% |
Average risk | 1% - 1.66% | 66.5% | 71% | 45.5% |
High risk | 1.67% - 6% | 33.5% | 29% | 43.3% |
Very high risk | > 6% | 0% | 0% | 0.4% |
"Potentially, by taking risk into account, we could mitigate the costs of using these different [imaging] techniques by applying them to women at higher risks or otherwise applying them to all women, but screening women ... at lower risk less frequently," Payne said.
However, the study did not measure the accuracy of the BOADICEA risk score versus the individual variables.
AI and breast density
The role of breast density in at-risk women can also be calculated using artificial intelligence (AI). Automated breast density assessment uses AI to calculate density estimates, using breast thickness and x-ray attenuation.
However, studies have shown a lack of strong correlations in density assessment across imaging modalities. In a second presentation at ECR, Samperna addressed these gaps with his team's research, which aimed to make automated assessment across all modalities more consistent.
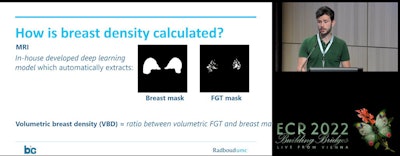
"When you're building bridges, remember to mind the gaps," Samperna said.
The researchers looked at data from 510 women with a total of 1,039 paired mammogram and MRI studies, with a maximum of one month between scans. They also used the medical center's in-house machine learning model that automatically extracts breast and fibroglandular tissue masks. These measures were combined with mammographic density measures.
Samperna et al found that they were able to match 81.1% of corresponding MRI studies perfectly in dense breast mammography cases. After adjusting for thresholds, 74.4% of studies showed an exact match between mammographic and MRI classes.
Samperna said these results can help bring easier personalized screening for women.