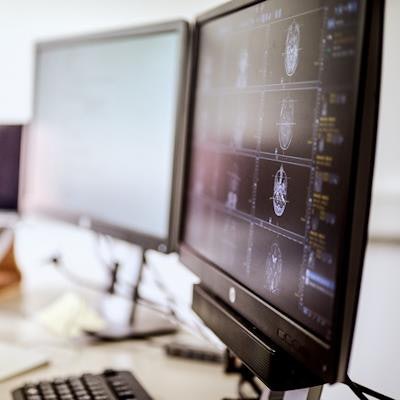
An artificial intelligence (AI) algorithm trained using a federated-learning approach yielded significant performance improvements in detecting pathology on brain MRI exams, according to research published August 25 in Nature Machine Intelligence.
After training a deep-learning model with their federated-learning method, a multi-institutional team from Germany led by senior author Shadi Albarqouni, PhD, of University Hospital Bonn and Helmholtz Munich found that the algorithm outperformed locally trained models in identifying pathologies such as multiple sclerosis (MS) and vascular lesions on brain MRI.
"Training the model on data from different centers contributes significantly to the fact that our algorithm detects diseases much more robustly than other algorithms that are only trained with data from one center," said co-author Dr. Benedikt Wiestler of Technical University of Munich's University Hospital Rechts der Isar in a statement from Helmholtz Munich.
In developing radiology AI algorithms, it can be very challenging to ensure that the model will be generalizable -- producing equivalent performance on data from institutions and equipment that are different from those it was trained on.
However, federated learning enables algorithms to be trained using data from multiple sites without having to share patient data. The technique has recently shown promise for producing high-performing algorithms for prostate MRI, hepatic CT scans, and predicting outcomes in COVID-19 patients.
In their study, the group from Helmholtz Munich, the University Hospital Bonn, and the University of Bonn sought to utilize federated learning and unannotated, normal brain MRI exams to develop a deep-learning algorithm to detect pathology. The team collaborated on the project with clinicians and researchers at Imperial College London, as well as the Technical University of Munich (TUM) and its University Hospital Rechts der Isar.
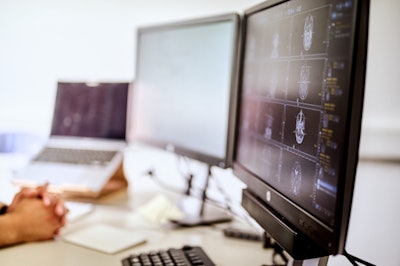
The researchers applied their federated learning concept -- called federated disentangled representation learning (FeDis) -- to normal brain MRI scans from four different sites and acquired on 3-tesla scanners from two different vendors (Philips and Siemens Healthineers). No local data was shared.
They then applied the resulting algorithm to 538 image volumes from six institutions to detect anomalies such as MS, vascular lesions (white-matter hyperintensities), and low-and high-trade tumors/glioblastoma. They found that the model trained with their FedDis federated-learning strategy produced significant improvements over models trained only on local data.
Performance on MRI segmentation of deep-learning model trained using federated learning | |
Improvement over locally trained models | |
Multiple sclerosis | 99.7% |
Vascular lesions | 83.3% |
Tumors | 40.5% |
"We found out that FedDis is especially beneficial for institutes that share both healthy and anomaly data, improving their local model performance by up to 227% for multiple sclerosis lesions and 77% for brain [tumors]," the authors wrote.
Ultimately, the researchers hope to make AI and healthcare affordable, according to Albarqouni.
"With our study, we have taken a step in this direction," he said in the Helmholtz Munich statement. "Our major goal is to develop AI algorithms, collaboratively trained at different, decentralized medical institutes, including those with limited resources."