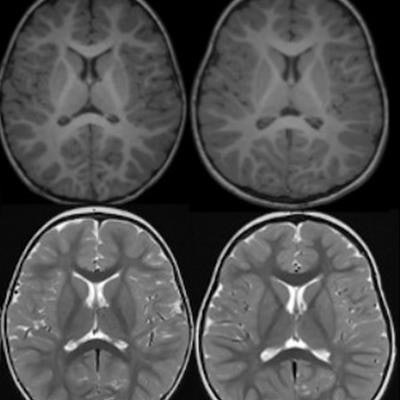
A deep-learning model helps radiologists accurately predict pediatric myelin maturation age on brain MRIs -- and it could help reduce the time needed to complete this task, a study published July 26 in Radiology: Artificial Intelligence has found.
Since healthy myelin maturation is key to healthy brain development, using AI to assess it could help clinicians intervene more quickly in children suspected of neurological conditions, according to a team led by Tugba Akinci D'Antonoli, MD, of University Children's Hospital in Basel, Switzerland.
"[It] is critical to assess myelin development to detect and treat developmental disorders in a timely manner," the group noted.
Myelin is a sheath that forms around nerves, including those in the brain and spinal cord, the team explained. Its maturation begins before birth and continues until a child is about three. Pediatric radiologists assess myelin maturation by tracking age-appropriate milestones and comparing brain MRI images to normal "templates." However, this task is tricky and requires years of expertise, the authors noted. That's why deep learning could help.
"Automating and objectifying [myelin maturation] could support and hasten the clinical decision-making process," they wrote.
For the study, the investigators developed three convolutional neural network models (2D, 3D, and 2D plus 3D) that they trained and validated using data from 833 children between the ages of zero and three to predict myelin maturation age based on radiologist assessments; of these, 275 had pathologies found on imaging. The patients underwent brain MRI exams between January 2011 and March 2021.
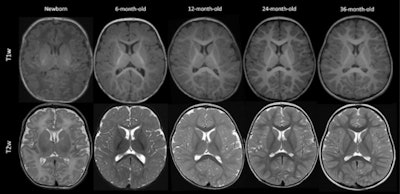
The group tested the three models on an internal dataset and on two external sets (these external sets included information from the National Institutes of Health pediatric brain MRI database and the Developing Human Connectome Project database). The team then evaluated the models' performance for assessing myelin age in months using mean absolute error (MAE) and Pearson correlation coefficients.
The researchers found that all three models performed comparably for estimating myelin age.
MAE in months for myelin assessment by deep-learning models | |||
Test set type | 2D | 3D | 2D plus 3D |
Cross-validation (710 subjects) | 1.53 | 2.06 | 1.63 |
Internal (123 subjects) | 1.43 | 2.55 | 1.77 |
National Institutes of Health (226 subjects) | 2.26 | 2.27 | 1.22 |
Developing Human Connectome Project (383 subjects) | 0.44 | 0.27 | 0.31 |
The three models also showed good agreement with the radiologist assessments for cross-validation, internal testing, and the NIH external test set, the team reported. What's more, the 2D plus 3D model outperformed a previously developed myelin assessment model reported in the literature with an MAE of 2.09, according to the researchers.
Not only does a deep-learning algorithm show promise for assessing myelin maturation but it could also mitigate interpretation differences among radiologists, according to the group.
"[Our study suggests that] a deep learning algorithm may be useful in predicting normal age-specific myelin development based on brain MRI," it concluded. "Such a pipeline could help overcome intra-and interobserver variability, while aiding the clinical decision-making process. In further steps, the same method could be used to identify patients with developmental delay and predict outcome."
The complete study can be found here.