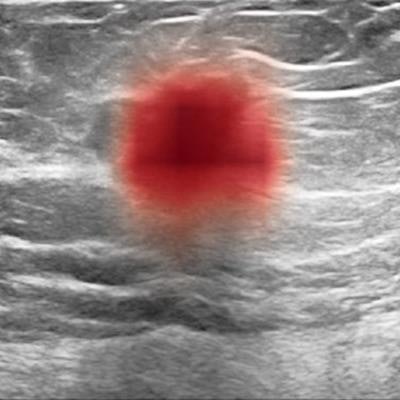
An artificial intelligence (AI) algorithm was able to significantly reduce false positives in breast ultrasound, potentially avoiding the need for one out of four biopsies in a study published online September 24 in Nature Communications.
Researchers from New York University (NYU) trained a deep-learning algorithm on nearly 290,000 breast ultrasound images. In the retrospective reader study, the model helped radiologists decrease false-positive rates by more than 37% and biopsy requests by more than 27% -- without affecting sensitivity. In addition, the algorithm demonstrated excellent generalizability on an external set of test data.
"Our study demonstrates how artificial intelligence can help radiologists reading breast ultrasound exams to reveal only those that show real signs of breast cancer and to avoid verification by biopsy in cases that turn out to be benign," said senior investigator Krzysztof Geras, PhD, of NYU Grossman School of Medicine, in a statement.
Although ultrasound offers value for detecting mammographically occult breast cancers -- especially in dense breasts -- the modality is also characterized by a high false-positive rate, according to the researchers. NYU co-first investigators Yiqiu "Artie" Shen, Farah Shamout, PhD, and Jamie Oliver and colleagues sought to address this problem by developing an AI algorithm.
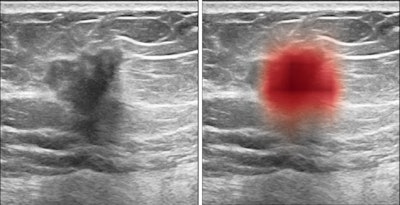
Training and validation of the deep neural network was conducted using a curated dataset of 288,767 breast ultrasound exams from 143,203 patients at NYU Langone Health between 2012 and 2019. In testing on 44,755 exams, the model yielded an area under the curve (AUC) of 0.976 for identifying breasts with malignant lesions and maintained high diagnostic accuracy across all age groups, mammographic breast densities, and ultrasound equipment manufacturers, according to the researchers.
Next, they compared the performance of the algorithm with that of 10 board-certified breast radiologists on an enriched sample of 663 exams from the internal test set. They also evaluated the results of a simulated hybrid prediction model that combined the predictions of the radiologists and the AI model.
Effect of AI on radiologists reading breast ultrasound | |||
Radiologists (average performance across 10 readers) | AI model | Hybrid model combining AI and radiologist predictions | |
AUC | 0.924 | 0.962 | Improved radiologist AUC by an average of 0.037 |
Specificity | 80.7% | 85.6% | 88% |
Sensitivity | 90.1% | 94.5% | Same as radiologists |
Biopsy rate | 24.3% | 19.8% | 17.6% |
Positive predictive value | 27.1% | 32.5% | 38% |
The hybrid model would have decreased the average radiologist's false-positive rate by 37.3%, while yielding the same number of false negatives or fewer as the radiologists, according to the researchers.
To assess the algorithm's potential generalizability, the researchers tested its performance on the 780 images in the Breast Ultrasound Images dataset from Baheya Hospital for Early Detection and Treatment of Women's Cancer in Cairo. The model yielded an AUC of 0.927.
"This highlights the potential of AI in improving the accuracy, consistency, and efficiency of breast ultrasound diagnosis worldwide," the authors wrote.
Although its initial results are promising, the software needs to be tested in clinical trials in current patients and real-world conditions before it could be routinely deployed, the group noted. Geras also plans to refine the AI software by including additional patient information such as a woman's added risk from having a family history or genetic mutation tied to breast cancer.
"If our efforts to use machine learning as a triaging tool for ultrasound studies prove successful, ultrasound could become a more effective tool in breast cancer screening, especially as an alternative to mammography, and for those with dense breast tissue," added study co-investigator Dr. Linda Moy in the statement.