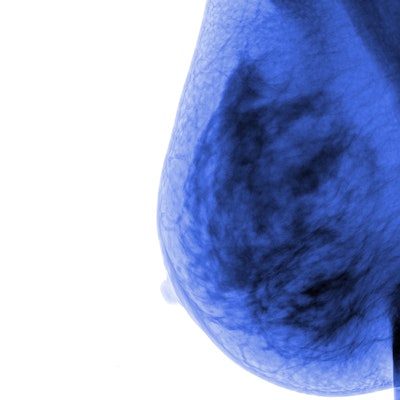
General radiologists and breast imaging subspecialists can both benefit significantly from reading digital breast tomosynthesis (DBT) studies concurrently with artificial intelligence (AI) software, reported researchers from the University of Pennsylvania and computer-aided detection (CAD) firm iCAD.
In a study involving more than 20 readers, the researchers found that concurrent use of AI during image interpretations resulted in better diagnostic performance for general radiologists and breast imaging subspecialists, with the general radiologists achieving the largest gains. What's more, reading times were cut approximately in half for both groups of radiologists.
"The study showed that performance improved with AI for both general radiologists and breast subspecialists, and interestingly, it showed that the general radiologists with AI performed [similarly] to the breast subspecialists without AI," said Dr. Jeffrey Hoffmeister, a vice president and medical director at iCAD and senior author of the study.
He shared the results on behalf of first author Dr. Emily Conant of the Hospital of the University of Pennsylvania and colleagues in a presentation at the recent Society for Imaging Informatics in Medicine (SIIM) annual meeting in Denver.
To assess the outcomes from concurrent use of AI with DBT separately for breast subspecialists and general radiologists, the researchers conducted a multireader, multicase study involving 24 readers and 260 DBT cases. The readers included 13 breast imaging subspecialists who devoted 75% or more of their time to breast imaging, as well as 11 general radiologists who spent less than 75% of their time reading breast imaging exams. All, however, had interpreted more than 500 DBT exams in the last two years.
Of the DBT cases, 65 were biopsy-proven as cancer and 65 were biopsy-proven as benign. The radiologists -- who had a range of experience from one to 34 years in clinical practice -- read each exam with and without AI, with at least four weeks in between reading the same case, according to the researchers. The interpretation order was randomized, and the radiologists were blinded to the patient's history and prior images. Reading time was calculated from the time the reader began reviewing images to the time the reader determined if the case had any suspicious lesions.
Developed by iCAD, the deep learning-based software used in the study detects both soft tissue and calcific lesions on DBT slices, Hoffmeister said. It also provides "certainty of finding" scores on both a lesion and case level.
Impact of AI software on performance and reading times of DBT | ||||
General radiologists without AI | General radiologists with AI | Breast imaging subspecialists without AI | Breast imaging subspecialists with AI | |
Area under the curve | 0.758 | 0.84 | 0.826 | 0.863 |
Case-level sensitivity | 71.5% | 84% | 81.7% | 85.9% |
Case-level specificity | 61.7% | 66.8% | 63.6% | 72% |
Mean reading times | 63.6 seconds | 32.7 seconds | 64.6 seconds | 28.5 seconds |
Reading time dropped by 55.9% for breast imaging subspecialists and by 48.5% for general radiologists, according to the researchers.