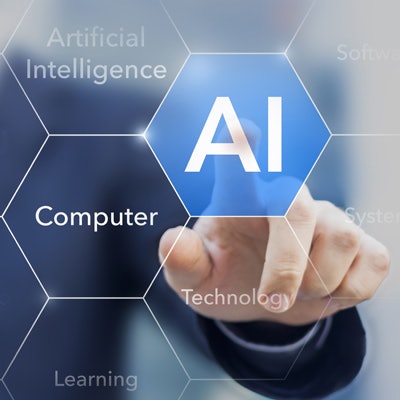
In theory, artificial intelligence (AI) should augment radiologists to improve patient outcomes. But will AI work this way in real life? Researchers shared insights on AI for breast imaging during an August 11 panel at the International Society for Magnetic Resonance in Medicine conference.
The presenters discussed their work on the use of deep learning and radiomics to predict cancer treatment outcomes and distinguish between cancerous and benign lesions. One of the most common lessons for the researchers was that more data isn't always better when it comes to AI.
"You would assume that having more features could improve prediction, but in reality, this can lead to overfitting when trying to train a predictive model," noted presenter Gabrielle Baxter, a doctoral student at the University of Cambridge radiology department, who has created an AI model to predict chemotherapy response in patients with breast cancer.
1. Larger area of focus may reduce resolution
In one presentation, Dr. Jiejie Zhou detailed how her team used deep learning to train an algorithm to accurately identify malignant breast tumors from dynamic contrast-enhanced (DCE) MRI scans. The team learned that having a wider area of focus quickly stifled their algorithm's accuracy.
Zhou, a radiologist at Wenzhou Medical University's First Affiliate Hospital in China, and colleagues created an algorithm to extract quantitative features from DCE-MRI images. They then used a deep-learning neural network to classify whether the peritumor tissue was benign or malignant.
Her team compared the neural network's diagnostic accuracy when paired with different bounding boxes -- squares that outline the area of interest around a tumor. The box sizes ranged from just outlining the tumor to expanding to up to twice the tumor size.
The neural network performed best when paired with the smallest bounding box that contained both tumor and peritumor tissue. The combination achieved a sensitivity of 94%, specificity of 81%, and an accuracy of 89% in an independent set of images.
But Zhou and colleagues also learned that the algorithm's diagnostic performance quickly worsened as the bounding box size expanded. The accuracy of the neural network's performance decreased from 89% at the smallest peritumor size to just 54% when the box doubled in size.
"As the size of the box increases, the performance becomes worse and worse, which might be in part due to lower input image resolutions into the neural networks and the information from the tumor is diluted," Zhou said.
2. Data from images can be redundant
In a similar project, Shizhan Gong described how the AI algorithm they trained using thousands of 3D MRI images didn't perform better when combining multiple models instead of just using the best performing one.
Gong, a master's of data science student at New York University (NYU), and his colleagues created a convolutional neural network that eliminated the need to delineate lesion margin, such as by using the bounding boxes described by Zhou. They achieved this goal by developing what they called a "doubly deep convolutional neural network" -- a neural network that can create 3D feature maps by breaking down and then putting back together data from 3D MRI images.
They trained and tested their neural network using 8,632 MRI exams from 6,295 patients at NYU Langone Health. Each examination produced 4 images for a total of 34,528 images.
Their neural network performed best when only using data from the second postcontrast MRI image subtraction image. This model achieved an area under the curve (AUC) of 0.73 for benign lesions and 0.85 for malignant lesions.
However, as Gong and colleagues continued to explore ways to improve the algorithm, they found accounting for multiple models or combining all of the image data had little effect on the AUC. The results showed that using more data doesn't necessarily translate to better results.
"We can conclude that from the perspective of our neural network the information in each image is redundant to a large degree," he said.
3. Quality is more important than quantity for features
In her presentation, Baxter discussed how her team at Cambridge used machine learning to analyze DCE-MRI scans in order to predict which patients with breast cancer were most likely to benefit from neoadjuvant chemotherapy before they even started treatment. They found using fewer features produced more accurate results.
Baxter and colleagues first used code to extract 384 features from the DCE-MRI images of 121 breast cancer patients. They then reduced the number of features down to 27 by only keeping features that differed significantly between patients who responded and didn't respond to treatment.
For their radiomics-based analysis, they trained an algorithm using 80% of the DCE-MRI images and tested the model using the remaining 20% of images. Their algorithm selected 21 of the 27 researcher-identified features as relevant for its computation.
Performance of DCE-MRI algorithm based on time point and features used | ||||
MRI images | No. of features | Sensitivity | Specificity | AUC |
All | 21 | 62% | 94% | 0.78 |
Third postcontrast image | 7 | 75% | 94% | 0.84 |
The model using the 21 algorithm-selected features and data from all five postcontrast MRI images achieved an AUC of 0.78. But when researchers narrowed in further, they realized a model using only seven of the most predictive features and the third postcontrast MRI image generated a much better performance, with an AUC of 0.85.
The results suggest that some features and timepoints may be more important than others. While Baxter concluded that radiomics can already help to predict which patients will experience a pathological complete response (pCR) to chemotherapy, she also said using better predictive features can further improve the accuracy.
"The addition of clinical and pathologic features, such as tumor subtype and hormone receptor status, ... have been shown to improve the performance of prediction pCR when used together with radiomic features," she said.