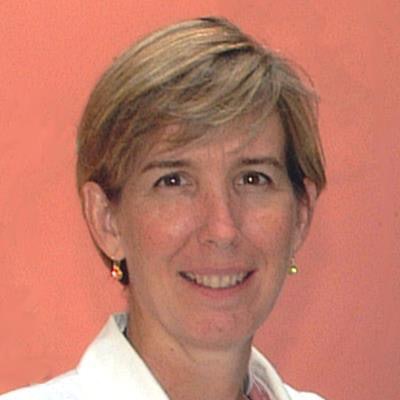
Larger datasets, more collaboration, and safety are a few improvements needed for artificial intelligence (AI) to work well alongside radiologists in screening mammography, according to research findings presented on March 6 at the AuntMinnie.com Spring 2021 Virtual Conference.
In the presentation, Dr. Emily Conant from the University of Pennsylvania cited multiple studies that indicate there is opportunity for more "useful" heterogeneous datasets collected by AI to localize cancers, as well as better transparency in collaboration to compare algorithms.
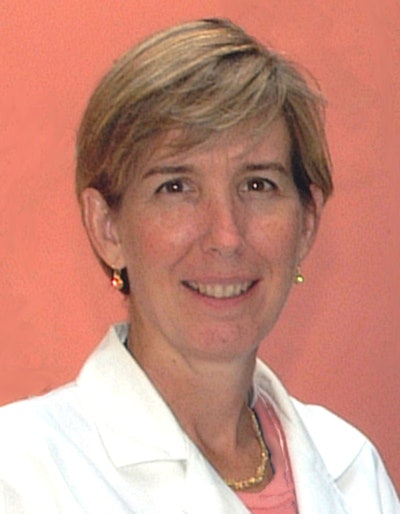
"AI is here for sure. I think we need to embrace it, validate it, and advance it," Conant said. "I really think we have the potential to become better radiologists, and most importantly, our patients will have better outcomes."
Conant also said there is reason for optimism for AI in breast imaging, with opportunities to improve accuracy, decrease variability in interpreting images, and improve efficiency in delivery of care.
"The bottom line, though, is all of that has to improve our patient outcomes," Conant said.
AI has grown from its computer-aided detection (CAD) roots since receiving U.S. Food and Drug Administration approval for screening mammography in 1998. By 2016, 92% of facilities were using CAD. However, older CAD methods did not show improvement in detecting cancer. Recent studies have shown that AI helps radiologists with better detection and diagnosis of breast cancer.
Conant led a study published in 2019 in Radiology that sought to improve accuracy and efficiency with concurrent use of AI with digital breast tomosynthesis (DBT). Twenty-four readers looked at 260 DBT cases, which included 65 cancer cases with 66 malignant tumors and 65 benign cases.
Her team's research showed that radiologists who had AI assistance were more accurate than radiologists interpreting images by themselves. Increases were seen in area under the curve (AUC) (5.7%), sensitivity (8%), and specificity (6.9%). Recall rate decreased by 7.2%, and reading time decreased by over half at 52.7%. All increases and decreases were statistically significant.
Conant said her study's limitations included being a retrospective, enriched reader study and that false negatives were not included.
"Because it was a small reader study, we definitely need to test this with a larger, more diverse dataset," she said.
In another study published in 2019 in Radiology, doctoral candidate Adam Yala from the Massachusetts Institute of Technology and his team found that their deep-learning model's AUC was 0.82. The model was trained on 238,271 digital mammograms.
It triaged 19% of studies as cancer-free and showed improvement in sensitivity, as well as a slight decrease in noninferior sensitivity.
AI seems to be better at detecting malignant masses, asymmetries, and distortions than radiologists alone. These include luminal B and triple-negative cancer types. It has also shown promise for improving the detection of interval cancers, according to research Conant cited in her presentation.
Conant said that the future of personalized breast cancer screening may be stratifying risk from data found by AI into different categories ranging from low to high. From there, doctors can customize care for patients.
However, many women seem skeptical of having AI alone interpret breast images.
In a survey study of 922 Dutch women published in January in the Journal of the American College of Radiology, 77.8% of women opposed standalone AI interpretations.
"That kind of makes sense," Conant said. "It's a little scary, that concept. We need a lot of validation and testing, and a larger dataset."
However, using AI triaging for a second read had more supporters. In the same study, 31.5% agreed with this method while 41.7% disagreed. The remaining survey respondents were undecided.
Meanwhile, 17% of respondents disagreed with having AI plus radiologists interpret images.
"It's very interesting," Conant said. "We have to think about our market and think about outcomes."