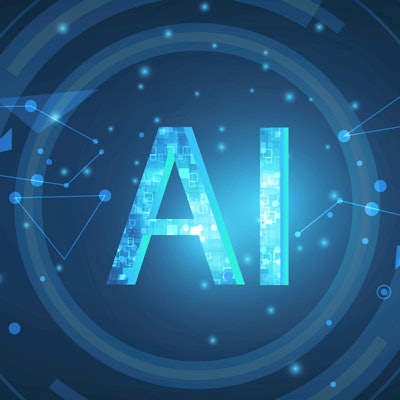
An artificial intelligence (AI) algorithm for screening mammography may be able to facilitate personalized breast cancer screening protocols for women, according to research published online September 7 in Radiology.
After training and testing a deep-learning algorithm on over 25,000 screening mammograms, researchers led by senior author John Shepherd, PhD, of the University of Hawaii Cancer Center in Honolulu found that their model predicted risk of breast cancer on future screening mammograms better than relying just on clinical risk factors including breast density.
"The results showed that the extra signal we're getting with AI provides a better risk estimate for screening-detected cancer," said Shepherd in a statement from the RSNA. "It helped us accomplish our goal of classifying women into low risk or high risk of screening-detected breast cancer."
On the downside, the AI algorithm didn't do as well for predicting interval cancer cases.
The researchers used a dataset of 25,096 digital screening mammograms acquired from 6,369 women at two clinics to train and test a deep-learning model to distinguish between women who did not develop breast cancer and those who went on to have an interval cancer or screening-detected cancer in subsequent years. Of the 6,369 women, 1,609 later developed screening-detected breast cancer and 351 had interval invasive breast cancer.
The researchers compared the performance of the algorithm on a hold-out set of 1,669 mammograms with a model based on clinical risk factors including BI-RADS density category, as well as a combined model. They used the C statistic to measure performance.
AI vs. BI-RADS density category for predicting future breast cancer | |||
BI-RADS density category | Deep-learning model | Combined model | |
Distinguishing future screening-detected cancers from control cases | 0.62 | 0.66 | 0.66 |
Distinguishing future interval-detected cancers from control cases | 0.71 | 0.64 | 0.72 |
"We conclude that the use of deep learning to identify mammography features combined with traditional clinical risk factors increases our ability to predict risk of screening-detected breast cancers," the authors wrote.
Instead of just relying on breast density to guide many management decisions and advising women to return next year for another screening, practices could use the AI model to categorize women with a negative screening into one of three risk pathways -- low risk of breast cancer, elevated screening-detected risk, or elevated interval invasive cancer risk -- for the next three years, according to the researchers.
"This would allow us to use a woman's individual risk to determine how frequently she should be monitored," Shepherd said. "Lower-risk women might not need to be monitored with mammography as often as those with a high risk of breast cancer."
What's more, the algorithm could also aid in deciding when to utilize additional imaging modalities. For example, women in the high-risk group who have dense breasts and have a higher risk for interval cancers may benefit from being monitored with supplemental imaging including MRI, ultrasound, and molecular imaging, according to the researchers.
"By ranking mammograms in terms of the probability of seeing cancer in the image, AI is going to be a powerful second reading tool to help categorize mammograms," Shepherd said.
In the next phase of their work, the researchers plan to replicate the study's results in Native Hawaiian and Pacific Islander women, as well as to extend the model to provide risk predictions for different grades of breast cancer.
As each individual woman has a different risk for breast cancer, it's possible that women should have personalized screening intervals and tailored options for supplemental screening, noted Dr. Min Sun Bae, PhD, of Inha University Hospital and School of Medicine in Incheon, South Korea; and Hyug-Gi Kim, PhD, of Kyung Hee University Hospital in Seoul, South Korea.
"The decision about breast cancer screening should be made through a shared decision-making process, enabling women to make an informed decision with their physicians based on individual risk assessment," Bae and Kim wrote in their accompanying editorial. "Further work is therefore needed to strengthen the risk prediction models to support personalized approaches to breast cancer screening."