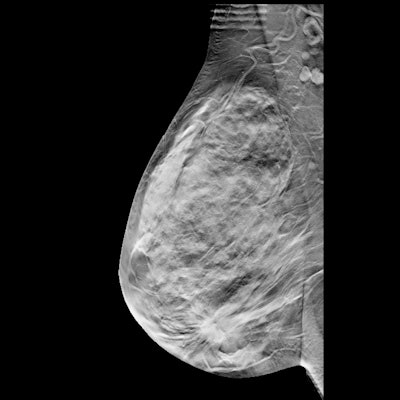
Artificial intelligence (AI) can help radiologists lessen their workload when reading digital breast tomosynthesis (DBT) images by nearly 40%, according to research published January 18 in Radiology.
A team led by Yoel Shoshan and Ran Bakalo from the University of Haifa in Israel developed an AI model that they say can filter out cancer-free cases and keep up with radiologists in terms of accuracy.
"Because 99.5% of screening examinations are cancer free, deploying such an AI system to optimize screening reads could be of substantial value," the group wrote.
The use of DBT is expected to grow worldwide, with previous research showing its ability to improve cancer detection and improve recall rate over conventional mammography. However, one downside to using DBT is it requires about twice the interpretation time as mammography, the researchers noted. This means more work for radiologists and higher costs for screening programs.
Previous research also suggests that AI can help save time and reduce workload for breast radiologists. Shoshan, Bakalo, and colleagues wanted to test the ability of their AI model to filter out normal DBT studies to reduce screening workload while improving diagnostic accuracy.
The team developed the model by training it in a cohort of 9,919 women with a total of 13,306 DBT exams. This was split into a training set of 3,948 women (804 cancers), a validation set of 1,661 women (182 cancers), and a test set of 4,310 women (453 cancers).
For the study, the researchers used the test set of data, which included a total of 5,182 DBT examinations performed between 2013 and 2018. The women in the test set had an average age of 60 years. The model was also used in a simulated workflow where it classified cancer-free examinations that could be dismissed from the screening worklist.
The model reduced screening workload by 39.6% with a sensitivity of 90.0%, while the radiologists had a sensitivity of 90.8% (p < 0.001), the researchers found. The model also filtered out cancer-free examinations, which led to a 25% decrease in the number of women who would have been recalled (6.9% versus 9.2%) (p < 0.001).
The AI system was also evaluated with a reader study of five breast radiologists reading the DBT mammograms of 205 women. The area under the curve (AUC) for the standalone AI was 0.84, compared with 0.81 for the average reader (p = 0.002).
The researchers envision their model affecting three different levels upon implementation in a clinical setting: reducing workload and fatigue in radiologists, improving workflow and further clearing the way for DBT in health systems, and reducing unnecessary recalls, stress, and radiation exposure for women.
"Future research should include prospective evaluation of our AI model, to assess the percentage of DBT examinations that would be removed from a prospective reading worklist, and to assess how readers perform when interpreting the remaining cases," the researchers wrote. "Future research should also evaluate generalizability to multiple DBT manufacturers."
In an invited commentary piece, Dr. Liane Philpotts from Yale University called for prospective studies to determine how AI's use will translate into real-world clinical settings, and she also noted that trusting AI could be a challenge for radiologists and patients.
"As radiologists, our relationship with AI will certainly evolve over time. Radiologists need to embrace and help mold the technology that is being developed," Philpotts wrote. "We can only hope continued efforts such as the study by Shoshan et al make progress toward improving both radiologists' workflow and confidence in interpretation as well as patient outcomes."