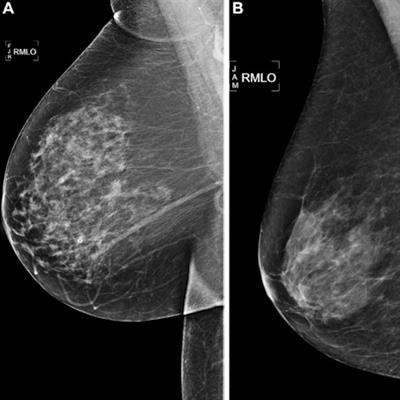
Mammography AI algorithms perform better than risk models for predicting breast cancer risk at zero to five years when using a negative screening exam, suggest findings published June 6 in Radiology.
Researchers led by Vignesh Arasu, MD, PhD, from Kaiser Permanente Northern California in Oakland also found that when AI was combined with risk models, breast cancer prediction improved.
"Our results imply that mammography AI algorithms alone may provide a clinically meaningful improvement compared with current clinical risk models at early time horizons, with further improvements in prediction when AI and clinical risk models are combined," Arasu and co-authors wrote.
Although several risk models are employed to guide breast cancer screening and prevention strategies, the researchers pointed out that they are only moderately successful at determining risk. However, AI's ability to extract imaging biomarkers may hold promise in predicting risk beyond just clinical factors used by current risk models. The authors noted that to their knowledge, there's a lack of data exploring AI's potential at predicting risk for shorter times over which risk is assessed.
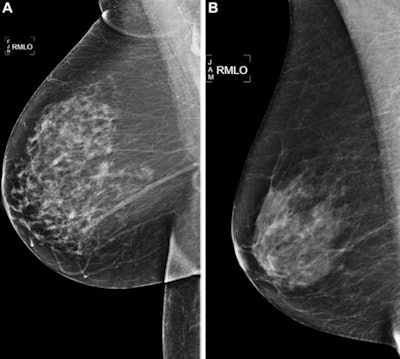
Arasu and colleagues wanted to compare five existing mammography AI algorithms and the Breast Cancer Surveillance Consortium (BCSC) risk model for predicting five-year breast cancer risk. Out of 324,009 eligible women, the team included data from 13,628 randomly selected women, 193 of whom had incident cancer. It also included data from an additional 4,391 women from the total eligible women who had incident cancer.
The researchers selected the following algorithms for use in the study: Mirai (Massachusetts Institute of Technology), MammoScreen (Therapixel), ProFound AI (iCAD), Mia (Kheiron), and GMIC (New York University).
The researchers found that the algorithms had higher time-dependent area under the curve (AUC) values than the BCSC model when it came to predicting risk of incident cancers at zero to five years.
Comparison between AI, BCSC risk model | |||
BCSC model | AI algorithms (range) | p-value | |
AUC | 0.61 | 0.63-0.67 | < 0.0016 |
However, the team also found that when the model and AI algorithms were combined, the time-dependent AUCs were higher than the range of AI algorithms alone, achieving an AUC ranging between 0.66 and 0.68 (p < 0.0016).
"Continued strong predictive performance... is surprising and suggests that AI is not only identifying missed cancers but may identify breast tissue features that help predict future cancer development," the group wrote.
The study authors suggested that larger sample sizes could further validate AI's performance compared to risk models. They added that AI can be a powerful way to stratify women for clinical considerations that need shorter time horizons, such as risk-based screening and supplemental imaging.
"The impact of AI models on clinical decisions requiring risk prediction beyond five years requires further study in cohorts with longer follow-up," the authors wrote.
You can read more about their findings here.