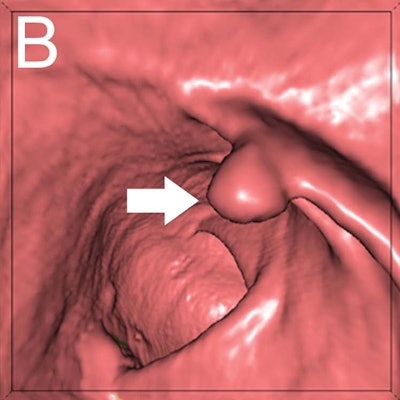
An artificial intelligence (AI) algorithm yielded a high level of accuracy for distinguishing between benign and precancerous polyps on CT colonography (CTC) exams, according to research published online February 23 in Radiology.
In a proof-of-concept study, a team of researchers led by Dr. Sergio Grosu of Ludwig Maximilian University of Munich in Germany trained a machine-learning model to characterize colon polyps based on radiomics analysis. In testing on an external dataset, the algorithm achieved an area under the curve (AUC) of 0.91.
"External validation of the technique in a large North American multicenter trial patient cohort demonstrated robustness and reproducibility of our method, despite data sets acquired by various scanner types and heterogeneous CT colonography imaging protocols," the authors wrote.
In colorectal cancer screening, CTC can perform comparably to colonoscopy for detecting polyps and can even visualize the portions of the colon that can't always be evaluated on colonoscopy, according to the researchers.
"But CT colonography does not enable a definite differentiation between benign and premalignant polyps, crucial for individual risk stratification and therapy guidance," the authors wrote.
The researchers sought to make up for this shortcoming with machine learning-based analysis of CTC radiomics. They developed their random-forest classification model using a training set of 107 colorectal polyps from 63 patients that included 169 manual segmentations on the CTC images. Polyps were classified as either benign (hyperplastic polyp or regular mucosa) or premalignant (adenoma) based on histopathology.
After applying 22 image filters, these manual segmentations were then used to extract quantitative image features, including 68 image texture features, 14 shape features, and 18 gray-level histogram statistics. This yielded a total of 1,906 feature-filter combinations, according to the researchers.
The researchers assessed the model's classification performance and generalizability on datasets from a North American multicenter CTC screening trial available from the Cancer Imaging Archive. This set of cases comprised 77 polyps in 59 asymptomatic patients with an average risk of colorectal cancer and included CTC exams acquired on CT scanners from several different vendors and with varying protocols.
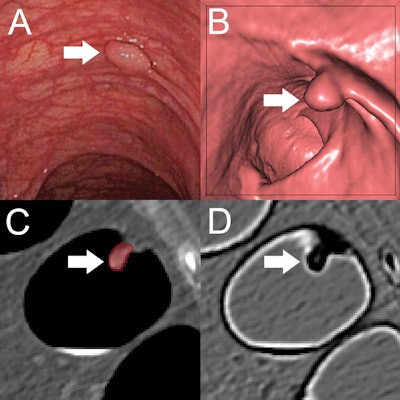
Their algorithm yielded a high level of performance on this external test set:
- AUC: 0.91
- Sensitivity: 82%
- Specificity: 85%
The authors also noted that the algorithm produced an AUC of 0.87 in polyps sized 6-9 mm and 0.90 in polyps sized 10 mm or larger. With a relative importance of 3.7%, first-order gray-level histogram statistics was the most important radiomics feature in the model's decision-making, according to the researchers.
"Adding machine learning-assisted image analysis to conventional, radiological image reading could further improve the clinical significance of CT colonography-based colorectal cancer screening by allowing for a more precise selection of patients eligible for subsequent polypectomy," said Grosu in a statement from the RSNA. "This method could be used routinely as a second reader in all CT colonography examinations in the distant future."
Prospective studies on a larger pool of patients are now needed to further examine the algorithm's performance and improve its results, according to the researchers.
"Further refinement of the machine learning-based image analysis is necessary to achieve higher precision in polyp differentiation as well as workflow optimization for better applicability in clinical routine," Grosu said.