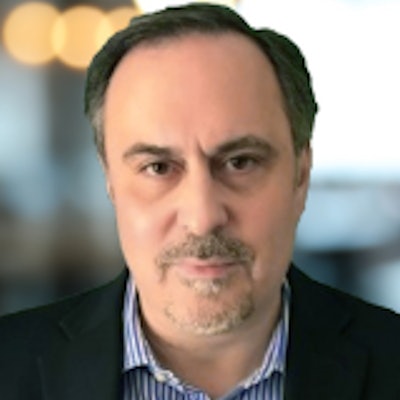
It wasn't that long ago that artificial intelligence (AI) proponents perpetuated the notion of AI marketplaces, believing that they provide a more straightforward way to purchase the technology that would soon lead to widespread adoption in hospital systems. But acquiring AI algorithms has never been the most challenging aspect of AI adoption.
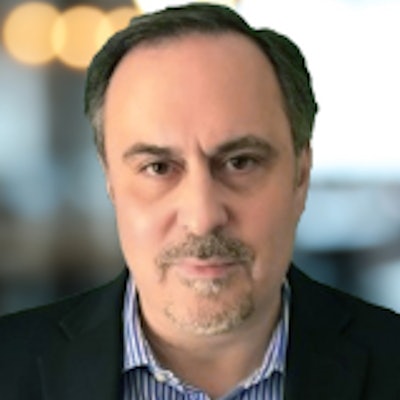
Industry surveys indicate that virtually all healthcare leaders have an AI strategy. However, physicians have cited a lack of trust in AI and the lack of a satisfying user experience as reasons for slow adoption. That's why interoperability is crucial as health systems seek to realize AI's clinical and financial benefits.
Radiology is a perfect example of this. Today, most radiology departments with AI-enhanced imaging applications have less-than-ideal workflow integration. Standalone AI solutions that require physicians to swivel to a different screen -- and worse, log in to separate systems -- force the physician to be the human data interoperability layer.
Enterprise AI platforms that deliver intersystem interoperability into physicians' current workflows are an excellent initial step. But interoperability is only part of the solution.
Radiologist buy-in
Physicians want confidence that AI insights are clinically valid and useful, but trust is difficult to earn when algorithms are viewed as black-box enigmas. An article in a peer-reviewed journal demonstrating the efficacy of a particular AI algorithm could provide a radiologist with some confidence that the datasets on which the algorithm was built are valid.
But a journal article speaks more to the general value of a specific AI application, not how it will translate to the radiologist's own use cases. A radiologist also wants to understand what data was used to build the algorithm, how the algorithm was validated, and, in particular, if the algorithm helped them improve diagnostic accuracy.
Today, many algorithms are developed using complex techniques that can obscure how the AI insights are derived and make them challenging to interpret. Moreover, these algorithms only allow clinicians to accept the AI recommendation or reject it -- with no opportunity to interact with the inputs or outputs. Suppose the algorithm delivers too many false-positive insights, and the physician continually rejects them. In that case, it won't be long before that algorithm is abandoned.
There are, however, algorithms that invite physician interaction. These algorithms and the associated alerts can be fully integrated into the radiologist's worklist, PACS, and integrated advanced visualization software. If the AI interpretation differs from the radiologist's, the radiologist can interrogate the finding and edit the results. He or she can see and understand how the AI input was derived and interact with the results at each interpretation step.
Trusting the result of AI is easier when the granularity of the AI process and workflow align with the radiologist's own interpretive process.
The right AI
While AI is well-suited to solve for specific clinical workflows like neurovascular emergencies or cardiac surgical procedures, its ability to solve some of the more annoying workflow gaps is where it can really make a difference in the quality of a radiologist's day and output. For instance, AI can be the departmental workhorse by correcting wrongly assigned series descriptions to different imaging modalities.
Today, up to 15% of these series descriptions are inaccurately entered. As a result, the proper imaging routing, processing, and display protocols cannot be initiated. An algorithm built to read and understand the anatomical content of the images and assign the correct series description has the potential to deliver substantial time savings to clinicians and supporting staff.
That doesn't even account for how imaging-based AI insights can be leveraged outside the walls of radiology. When aligned to a larger enterprise strategy, AI algorithms can, for instance, improve how physicians leverage electronic medical record (EMR) data or drive extended-care team workflows at the patient's bedside or, perhaps one day, even in their home? In this scenario, a platform-based interoperability strategy becomes even more essential.
The radiologist's place
With their reputation as early adopters of new technology, radiologists are perhaps best-suited to guide a hospital in developing a long-term, enterprise-wide AI strategy. In addition, with a significant percentage of patient encounters involving imaging studies, radiologists are gaining real-world experience integrating AI into their workflows and demonstrating the utility of AI to clinicians in other specialties.
For example, clinicians working in a 3D lab may have 10 cases for surgery prep, with work taking about an hour for each case. In this scenario, AI can handle the preprocessing and allow clinicians to quickly edit those results, increasing their productivity.
Consider how that kind of time-saving impact could affect entire hospital systems. It could allow greater speed to insights, faster interventions, and improved treatments. And it all starts with the radiology department.
Sinan Batman is the CTO and chief product management officer of TeraRecon, a ConcertAI company.
The comments and observations expressed are those of the author and do not necessarily reflect the opinions of AuntMinnie.com.