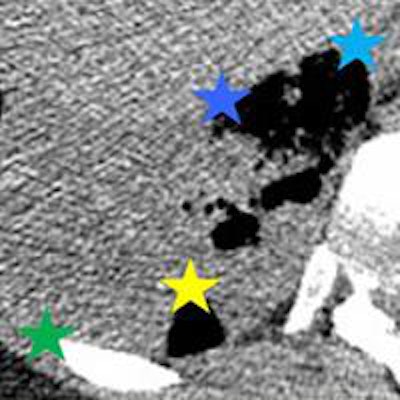
Perfusion CT is emerging as a useful way to assess the treatment of lung cancer patients, but respiratory motion can cause problems with images. Fortunately, researchers from California and Pennsylvania have discovered a motion correction algorithm that can help.
Investigators from the two institutions hypothesized that a motion correction algorithm could lead to more accurate perfusion CT follow-up of tumors in patients with lung cancer. The study revealed that both intra- and interobserver variability in interpreting the images improved markedly with use of the motion correction algorithm.
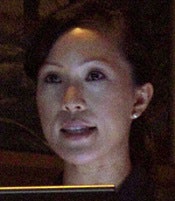
"Respiratory motion is an important compromising factor in measuring lung cancer blood flow, and use of an original motion correction method significantly improves reproducibility of measurements of lung cancer at perfusion CT," said Dr. Lisa Chu from the University of California, Davis (UC Davis), in a presentation at the RSNA 2015 meeting earlier this month.
How RECIST falls short
Following up lung cancer tumors with perfusion CT is invaluable for measuring response to newer treatments because it measures vascularity directly, a feature that can change faster than tumor size, Chu said. UC Davis currently uses Response Evaluation Criteria in Solid Tumors (RECIST) to evaluate treatment response, but the technique isn't optimal for today's lung cancer treatment regimens.
"RECIST was originally developed to assess tumor response to cytotoxic therapy," Chu said. "However, antiangiogenic drugs, which are more commonly being used, can change tumor vascularity long before changing tumor size, so RECIST may not be as sensitive in assessing tumors that are treated with antiangiogenic drugs."
However, respiratory motion in CT perfusion images is a constant problem because it requires the patient to hold his or her breath for more than 40 seconds.
"Patients with lung cancer already have a lot of trouble holding their breath, so this is a problem," Chu said. "Respiratory motion artifacts can lead to substantial misregistration. Currently, at our institution, we have no automated method to correct for respiratory motion, so we had to come up with our own."
The study conducted at UC Davis and the University of Pittsburgh explored whether motion correction could improve the reproducibility of blood-flow measurements of perfusion CT in lung cancer. The researchers decided that lower levels of intra- and interobserver variability would reflect greater accuracy in blood-flow measurements, so they developed a study protocol focusing on that metric.
The investigators examined 20 patients with nonresectable, biopsy-proven non-small cell lung cancer at the time of their scheduled CT scan. All had at least one lung tumor 1 cm or larger. Following an injection of 30 mL of contrast, the tumor was scanned continuously with perfusion CT on a 64-detector-row scanner for 50 seconds at 5 mAs and 100 kVp, acquiring 64 images at 1.25-mm slice thickness. Images were reconstructed using 30% adaptive statistical iterative reconstruction (ASIR).
ImageJ and Matlab programs were used to create thin-slice images of the tumor, and both rigid and nonrigid registration methods (MedInria open-source software) were applied, with the investigators lining up image landmarks and correcting them for motion. Next, they converted the thin-slice images back into thick slices in Matlab and uploaded the motion-corrected images to a workstation (Advantage, GE Healthcare), Chu said.
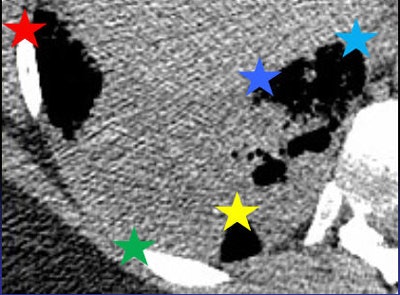
Commercially available perfusion analysis software was used to analyze the tumors from their dual-source blood supply in the pulmonary and bronchial arteries, creating blood-flow maps. Two readers analyzed each image before and after motion correction, and intraobserver and interobserver variability of each method was calculated.
Less variability
There was less variability between the readers interpreting images after motion correction was applied, the researchers found. Variability was measured in terms of limits of agreement, in which a narrower range indicates less variability.
Variability with motion correction | ||
Before motion correction | After motion correction | |
Intraobserver variability | ||
Reader 1 | -84.4% to +65.3% | -33.8% to +30.33% |
Reader 2 | -151% to +95.7% | -48.5% to +36.0% |
Interobserver variability | ||
Readers 1 and 2 | -168.2% to +153.8% | -17.3% to +25.3% |
For reader 1, intraobserver reproducibility improved from -84.4% to +65.3% before motion correction to -33.8% to +30.33% after motion correction (r = 0.86 and 0.97, before and after motion correction, respectively; p < 0.0001 for both).
Intraobserver reproducibility improvement for reader 2 was even more dramatic, narrowing from -151% to +95.7% before motion correction to -48.5% to +36.0% after motion correction (r = 0.87 and 0.95, before and after motion correction, respectively; p < 0.0001 for both).
At the same time, combined interobserver reproducibility between reader 1 and reader 2 improved from -168.2% to +153.8% before motion correction to -17.3% to +25.3% after motion correction (r = 0.65 and 0.97, before and after motion correction, respectively; p < 0.0001 for both).
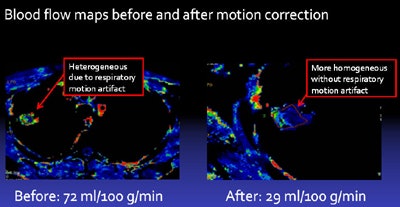
Based on the results, the researchers concluded that motion correction significantly improves the reproducibility of CT perfusion estimates of tumor blood flow in lung cancer, Chu said.
She acknowledged several limitations of the study, including its small sample size and the more than an hour of processing time required for each case. Also, the reproducibility results were not stratified by tumor size or location, and this could affect the degree of improvement because motion artifact is potentially greater at the lung bases, she said.
Respiratory motion significantly compromises blood-flow measurements in lung cancer and the problem is greatly reduced using a motion correction algorithm, Chu said. Still, owing to the long processing times of more than an hour for each case, the technique "is not practical" for widespread clinical use at this time, she added.