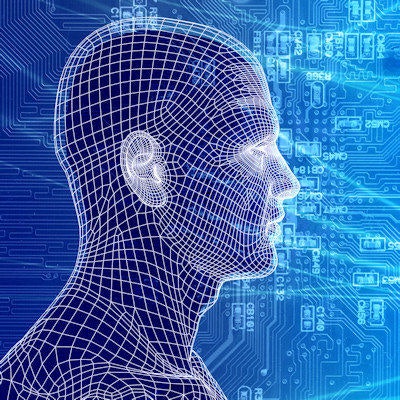
While some barriers still need to be cleared, machine-learning technologies are rapidly being developed for a variety of clinical applications in medical imaging and are poised to significantly affect radiology practice and patient care, according to an article published online June 26 in Radiology.
In a commentary, a team of authors led by Dr. Garry Choy of Harvard Medical School described how machine learning offers the promise of enhancing radiology workflow steps such as order scheduling and patient screening, clinical decision-support systems, detection and interpretation of findings, and postprocessing and dose estimation, as well as radiology reporting. It also could facilitate more personalized medicine.
"For the foreseeable future, widespread application of machine-learning algorithms in diagnostic radiology is not expected to reduce the need for radiologists," the authors wrote. "Instead, these techniques are expected to improve radiology workflow, increase radiologist productivity, and enhance patient care and satisfaction."
The authors highlighted 11 clinical applications for machine learning in radiology:
- Order scheduling and patient screening
- Image acquisition
- Automated detection of findings and features
- Automated interpretation of findings
- Automated clinical decision support and exam protocoling
- Postprocessing: Image segmentation, registration, and quantification
- Image quality analytics
- Automated radiation dose estimation
- Radiology reporting and analytics
- Automated data integration and analysis
- Automated correlation and integration of medical imaging data with other data sources
Development and clinical adoption of these applications will require overcoming a number of hurdles, though, including collecting high-quality ground truth data to train machine-learning algorithms, as well as formulating techniques that are diagnostically accurate, generalizeable, and interoperable between different IT systems and software applications, according to the authors. Workflow integration also remains an important issue.
"Vendors and researchers alike must aim to create platforms that will allow for continuous learning and upgrades of machine-learning algorithms," they wrote. "Machine-learning algorithms need to be updated continuously based on possible changes in the model through exposure to more data."
Looking into the future, machine learning shows potential for enabling personalized healthcare, according to the authors. By extracting knowledge from the large amount of data in electronic medical records, machine learning can produce outputs that could be used for individualized outcome prediction analysis and clinical decision-making.
"This could make way for personalized medicine (or precision medicine), in which individual variability in genes, environment, and lifestyle factors for each person is taken into account for disease prevention, treatment, and prognostication," they wrote.
Machine-learning approaches that can interrogate a wide spectrum of data -- sociodemographic, imaging, clinical, laboratory, and genetic -- could personalize healthcare far beyond what's possible with imaging applications alone, according to the authors. Indeed, imaging data will be linked more readily in the future to nonimaging data in electronic medical records and other large datasets.
"Deep learning, when applied to electronic medical record data, can help derive patient representations that may lead to clinical predictions and augmentation of clinical decision-support systems," they wrote.