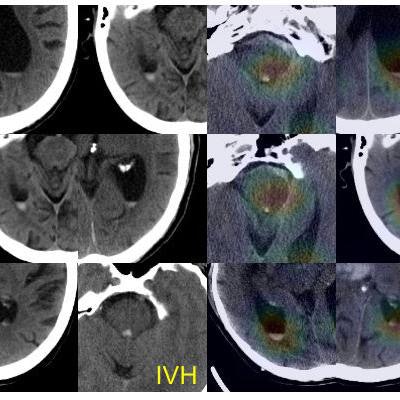
Researchers have developed a new artificial intelligence (AI) algorithm designed to address two of the biggest challenges in imaging AI: its "black box" nature and the need for large amounts of image data to train the models, according to a study published online December 17 in Nature Biomedical Engineering.
Researchers from Massachusetts General Hospital (MGH) in Boston used just over 900 cases to train a deep-learning algorithm how to detect intracranial hemorrhage (ICH) and classify all five of its subtypes on unenhanced CT exams. They found that their model achieved higher sensitivity than most of the radiologists in their study.
In addition, the system "explains" its findings by highlighting on an "attention map" the important image regions used to make its predictions. It also shares the basis of its predictions by displaying similar results from training cases.
"Our approach to algorithm development can facilitate the development of deep-learning systems for a variety of clinical applications and accelerate their adoption into clinical practice," wrote the group led by doctoral candidate Hyunkwang Lee and Dr. Sehyo Yune.
A potentially fatal condition
Brain hemorrhage is a potentially fatal emergency condition and must be ruled out prior to initiating treatment in any patient with symptoms or signs of stroke, Yune said. As a result, radiologists are always expected to determine the presence or absence of bleeding when interpreting a brain imaging study.
"An automated, sensitive, and understandable model that reliably detects intracranial hemorrhage can ensure patient safety by preventing delayed or missed diagnosis of ICH," she said. "It can also expedite the treatment pathway for patients who need revascularization therapy, which is critical in preserving neurological functions in ischemic stroke but must be avoided in hemorrhagic stroke."
To help tackle this problem, the MGH researchers developed a system comprised of four ImageNet-pretrained deep convolutional neural networks (VGG16, ResNet-50, Inception-v3, and Inception-ResNet-v2), an image preprocessing pipeline, an atlas creation module, and a prediction-basis-selection module.
Deep-learning obstacles
While working on the various deep-learning models for medical image analysis, the researchers encountered two particularly big obstacles: the challenge of collecting high-quality "big data" and the black box nature of algorithms, Lee said. They realized early on that the performance of the model was directly associated with the quality of labels on the images used for training. As a result, they elected in their study to improve the quality of the training data, rather than the quantity.
Using 904 noncontrast head CT exams from their institution's PACS, five subspecialty-trained and board-certified neuroradiologists labeled each of the 2D axial images in consensus as having no hemorrhage, intraparenchymal hemorrhage, intraventricular hemorrhage, subdural hemorrhage, epidural hemorrhage, or subarachnoid hemorrhage. Of the 904 cases, 704 were used to train the model; 100 cases with ICH -- including all subtypes -- and 100 cases without ICH were set aside as a validation dataset.
"This [training approach] was contrary to the common belief that bigger data is a prerequisite for developing a deep-learning algorithm, but we witnessed significant improvement of the model performance by using more carefully labeled data and applying techniques that are specifically developed for the application -- ICH detection from nonenhanced CT," Lee told AuntMinnie.com.
Yune noted that the inability of deep-learning models to "explain" their findings has been a universal concern that led many clinicians to remain dubious of the algorithms' clinical utility.
To address that issue, "I used my own experience during medical school and residency in detecting a condition from medical images; with a preliminary impression in mind, I looked up images from other cases that were confirmed with the diagnosis, and compared the similarity between the confirmed cases and the current case," she said. "So we came up with the idea to show an 'atlas' to allow clinicians to confirm or reject the model prediction (preliminary impression) by comparing a case with cases confirmed by experts. The explainability is the key for nonexpert users in determining the reliability of each model prediction, while the use of a relatively small dataset opens the door to many more applications for which 'big data' is difficult to collect or does not exist."
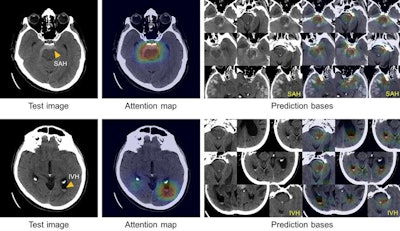
Model performance
In addition to assessing the algorithm's performance on the test dataset of 200 cases, the researchers also evaluated it on a prospective dataset of 79 cases with ICH and 117 cases without ICH that were collected consecutively from their institution's emergency department over a four-month period.
AI model performance in detecting ICH | ||
Retrospective dataset | Prospective dataset | |
Sensitivity | 98% | 92.4% |
Specificity | 95% | 94.9% |
Area under the curve | 0.993 | 0.961 |
Next, the researchers compared these results with those of five radiologists -- three residents and two subspecialty-certified neuroradiologists. The model performed comparably to the radiologists in the retrospective test set, but it delivered higher sensitivity in the prospective test set than all five radiologists.
In terms of classifying ICH subtype, the algorithm achieved an area under the curve (AUC) on the retrospective dataset that ranged between 0.92 (for epidural hemorrhage) and 0.98 (for intraparenchymal hemorrhage). On the prospective dataset, the model provided an AUC of 0.88 (for subdural hemorrhage) to 0.97 (for intraventricular hemorrhage).
Future work
The researchers are now in the process of testing their algorithm on a prospective, consecutive dataset of emergency department patients to assess its performance and generalizability, Lee said. The researchers have learned that the model's performance varies widely depending on the case mix and image quality of test datasets.
"We believe it is essential to understand how to interpret model predictions in each clinical setting, and the current study focuses on the real-world applicability," he said.