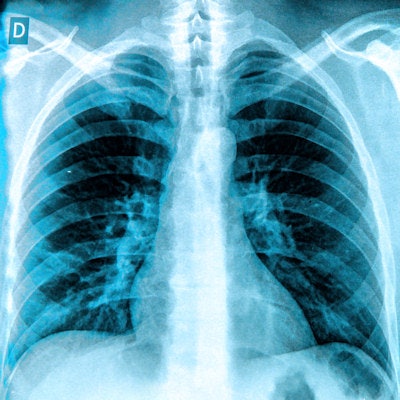
Researchers from Stanford University have released a massive dataset of more than 224,000 chest x-rays to be used for the development of artificial intelligence (AI) algorithms. The group has also launched a competition for developers of AI algorithms to test their models.
Called CheXpert, the database was released by Stanford's Machine Learning Group. It features 224,316 chest radiographs and radiology reports retrospectively gathered on 65,240 patients at Stanford Hospital's outpatient and inpatient centers between October 2002 and 2017.
Importantly, each radiology report is labeled as positive, negative, or uncertain for the presence of 14 specific observations. These structured labels were created for the images by an automated rule-based labeler developed by Stanford that uses natural language processing to extract observations from free-text radiology reports. This open-source automated labeler is also publicly available for download.
"Automated chest radiograph interpretation at the level of practicing radiologists could provide substantial benefit in many medical settings, from improved workflow prioritization and clinical decision support to large-scale screening and global population health initiatives," the group wrote on the CheXpert webpage. "For progress in both development and validation of automated algorithms, we realized there was a need for a labeled dataset that (1) was large, (2) had strong reference standards, and (3) provided expert human performance metrics for comparison."
Notably, Stanford is co-releasing CheXpert along with Medical Information Mart for Intensive Care (MIMIC)-CXR, another new public dataset of 371,920 chest x-rays associated with 227,943 imaging studies sourced from Beth Israel Deaconess Medical Center in Boston between 2011 and 2016. The Stanford labeler was also used to create structured labels for the images in the MIMIC-CXR dataset.
After developers train their chest radiograph algorithms, they can enter a competition to see if their models perform as well as radiologists for detecting different pathologies. Participating teams will be able to submit executable code for official evaluation on a test of 500 studies from 500 patients that had not previously been seen by the models.
The Stanford group said that each of the 500 studies was individually annotated by eight board-certified radiologists, who classified each of the 14 observations as present, uncertain likely, uncertain unlikely, and absent. All annotations of "present" or "uncertain likely" were considered to be positive results, while all "absent" or "uncertain unlikely" findings were treated as negative results. A majority vote of five radiologist annotations served as ground truth for the study, while the other three radiologist annotations were used to benchmark radiologist performance, according to the group.
Current algorithm scores on the test set will be displayed on a leaderboard on the competition's webpage beginning in February. The researchers noted that, on a per-observation basis, their own baseline chest radiograph interpretation model achieved higher performance than the three radiologists individually for cardiomegaly, edema, and pleural effusion, but not compared with their majority vote. All three radiologists performed better than the model for atelectasis, while the model bested two of the three radiologists for the observation of consolidation.