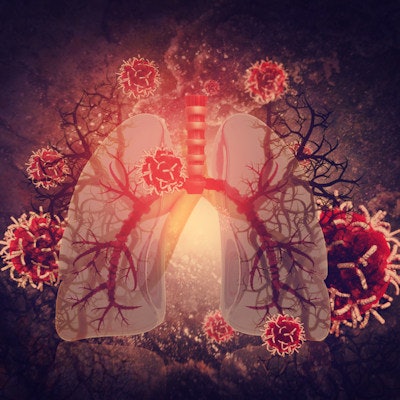
An artificial intelligence (AI) algorithm for chest x-rays that was tested in real-time in the emergency department (ED) could improve the quality of care for patients suspected to have pneumonia, according to research presented on September 30 at the European Respiratory Society International Congress in Madrid.
Using a fine-tuned version of the CheXpert AI model developed at Stanford University in California, a team of researchers led by Dr. Nathan Dean of Intermountain Medical Center in Salt Lake City found that the algorithm performed comparably with radiologists and only took 10 seconds to process the radiographs.
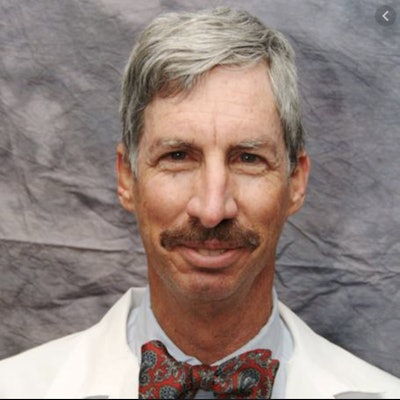
"CheXpert is going to be faster and as accurate as radiologists viewing the studies," said Dean, section chief of pulmonary and critical care medicine at Intermountain, in a statement. "It's an exciting new way of thinking about diagnosing and treating patients to provide the very best care possible."
Patients presenting to EDs with suspected pneumonia typically receive a chest x-ray. However, it can take 20 minutes or more for these studies to be read by a radiologist, a delay that can impede patient care, according to the researchers. In addition, ED physicians often find it challenging to understand the unstructured language used by radiologists in interpreting shadows on x-rays, Dean said.
Furthermore, Intermountain EDs process radiology reports through Cerner's natural language processing (NLP) software, which analyzes the reports and then feeds information into the institution's ePNa clinical decision-support application utilized as part of Intermountain's typical pneumonia care. This process has been error-prone, however, according to Dean and colleagues from Intermountain and Stanford.
As a result, they sought to determine if CheXpert -- an AI model trained by the Stanford Machine Learning Group on 188,000 chest radiographs -- could improve the speed and accuracy of identifying the key chest imaging findings required for diagnosing and treating pneumonia. After first fine-tuning the model's performance on 6,973 ED chest images from the local population at Intermountain, the Stanford and Intermountain researchers then tested it on the chest radiographs of 461 Intermountain ED patients, 66% of whom had pneumonia.
Three Intermountain radiologists independently evaluated the images for radiographic evidence of pneumonia, single-lobe versus multilobe pneumonia, and the presence of parapneumonic effusion. They categorized those findings as likely, likely-uncertain, unlikely-uncertain, or unlikely to have pneumonia. The researchers found there was disagreement among the radiologists in more than half of the cases.
They then compared performance of CheXpert with the consensus interpretation among the three radiologists -- the gold standard for the study.
Performance of CheXpert for detecting key pneumonia findings | |
CheXpert (area under the curve) | |
Detecting pneumonia | 0.838 |
Differentiating between single lobe vs. multilobe pneumonia | 0.839 |
Detecting significant parapneumonic effect | 0.944 |
The CheXpert algorithm's results correlated well with the consensus interpretation of the three radiologists and also outperformed the NLP processing for all key pneumonia findings, according to the researchers. They said they now plan to deploy the CheXpert model for live use at select Intermountain Healthcare Hospitals this fall.