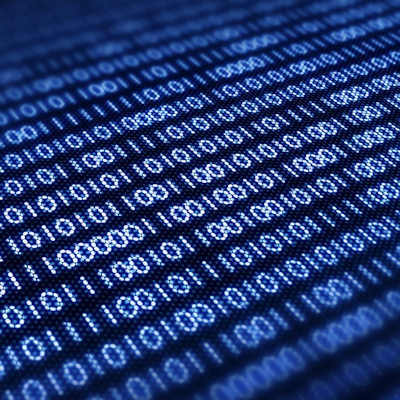
An algorithm based on natural language processing (NLP) was able to analyze radiology reports for text that recommended follow-up imaging, while a second algorithm determined whether patients followed the recommendations, according to an article published online March 12 in the American Journal of Roentgenology.
Using automated methods to assess adherence to imaging follow-up recommendations from two radiology departments, a team of researchers from Philips Healthcare, the University of Washington (UW) in Seattle, and Lahey Hospital and Medical Center (LHMC) in Burlington, MA, found that follow-up rates varied by location and imaging modality.
"Having the capability to identify patients who can benefit from patient engagement initiatives is important to improve overall adherence rates," wrote the team led by Thusitha Mabotuwana of Philips and UW. "Monitoring of follow-up adherence rates over time, and critical evaluation of variation in recommendation patterns across the practice can inform measures to standardize and help mitigate risk."
Radiologists often include recommendations for follow-up imaging in radiology reports; failure to comply with this advice can lead to poor patient outcomes, complications, and legal liability, according to the researchers. Following up on previous work developing NLP and machine learning-based algorithms for automatically matching imaging recommendations with a subsequent radiology exam, the researchers applied their models to extract radiology-related examination data from studies performed between June 2015 and July 2017 at UW and LHMC.
The UW dataset comprised 923,885 imaging exams, while the LHMC dataset included 763,059 exams. The researchers used a one-year period for detection of imaging recommendations and up to 14 months afterward for the follow-up exam to be performed.
The NLP algorithm, which offered 97.9% detection accuracy for identifying follow-up imaging recommendations in radiology reports, found that 11.4% of the reports at UW and 20.9% of the reports at LHMC had a recommendation for follow-up imaging. A machine learning-based matcher algorithm, which yielded 76.5% accuracy during testing, was then used to determine if the recommended follow-up exams were actually performed.
Adherence to follow-up imaging recommendations | ||
UW | LHMC | |
Computed and digital radiography | 47.1% | 45.8% |
CT | 53.5% | 51.7% |
Fluoroscopy | 53.9% | 30.1% |
Mammography | 60.1% | 86.3% |
MRI | 63% | 48.3% |
Nuclear medicine | 44.4% | 41.9% |
PET, including PET/CT | 49.9% | 36.7% |
Ultrasound | 50.2% | 63.2% |
Overall (excluding mammography) | 51.9% | 52% |
Overall (including mammography) | 55.1% | 68.6% |
"Our results suggest that the mean follow-up adherence rates at modality level vary between the two institutions, although mammography, MRI, CT, and ultrasound were the top four modalities by adherence rate at both institutions," the authors wrote.
New scalable techniques are needed to enable radiology administrators to easily identify opportunities to improve low follow-up imaging adherence rates, they noted.
"Validation of cases of missed follow-up examination and effective intervention will likely require a combination of computer-aided and human activities," according to the authors. "With the gradual transition to value-based healthcare, improving adherence to follow-up recommendations could be one of the ways radiology can provide more value to the referring physicians and contribute more toward the overall management of the patient."