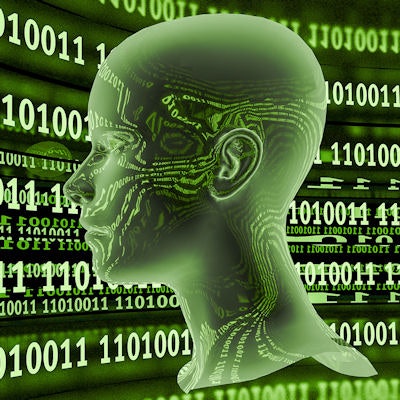
Careful stewardship of the integrity of big data is crucial to ensure that inherent biases contained in datasets used to train artificial intelligence (AI) algorithms don't make existing disparities in healthcare even worse, according to an article published online August 19 in npj Digital Medicine.
In a perspective article, a team of researchers reviewed the risks of biased data sources and offered some suggestions for addressing these challenges. The team included senior author Dr. Daniel Rubin of Stanford University and lead author Dr. Eli Cahan, who is from Stanford and New York University.
"Awareness of data deficiencies, structures for data inclusiveness, strategies for data sanitation, and mechanisms for data correction can help realize the potential of big data for a personalized medicine era," the authors wrote. "Applied deliberately, these considerations could help mitigate risks of perpetuation of health inequity amidst widespread adoption of novel applications of big data."
As has been the case in predictive AI algorithms developed in fields outside of medicine, algorithms in biomedicine have demonstrated racial biases, such as the prognostic models developed from the Framingham Heart Study and precision medicine protocols based primarily on European ancestral genotypes, according to the researchers. Although the algorithms are often blamed for these deficiencies, the authors believe the data used to train the algorithms bears greater responsibility.
"The concept of 'garbage in, garbage out' is of the utmost importance for medical algorithms trained on healthcare datasets and impacting patients downstream," they wrote.
The conventional paradigm for utilizing big data is deductive in nature, i.e., for clinical decision support such as determining whether or not to order a CT scan. However, a future model may harness big data's potential for inductive reasoning, according to the authors.
"This may be conceptualized as clinical decision questioning, intended to liberate the human predictive process from preconceived lenses in data solicitation and/or interpretation," they wrote. "Efficacy, representativeness, and generalizability are all heightened in this schema."
The authors recommended the following several strategies for ensuring the integrity of big data:
- Annotating training datasets with labeling metadata can heighten transparency by documenting intrinsic biases such as sampling imbalance.
- Redesigning data collection methods -- specifically relating to peripheral digital platforms -- can ensure data variety beyond volume alone. This could include deliberate outreach efforts by investigators to populations with poorer healthcare access. When outreach efforts can't feasibly be conducted, imputation of heterogeneity to datasets and utilization of federated methods can support data veracity through inclusion of diverse feature sets.
- Journals should adopt dataset quality standards and minimum thresholds of inclusiveness used for analysis.
- Transparency into the characteristics of datasets should be provided to practitioners attempting to interpret emerging studies.
"Mobilization of the technology itself in an inductive fashion can also support these appraisals," they wrote. "For example, methods like contrastive principal component analyses, which compare multidimensional enrichment patterns between datasets, are capable of visualizing ingrained data biases. Identification of the shortcomings of datasets offers one path to improving the utility of studies."