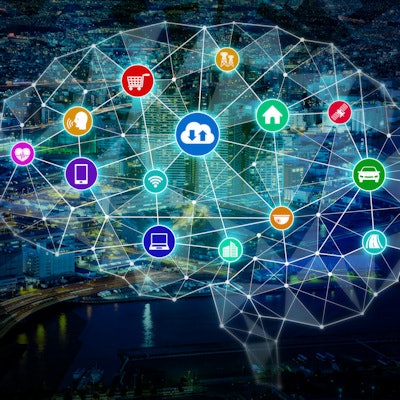
A new approach to training artificial intelligence (AI) algorithms on imaging data from multiple institutions can produce high-performing models -- while still preserving patient privacy, according to new research from graphics processing unit technology developer Nvidia and King's College London.
In a study presented October 14 at the Medical Image Computing and Computer Assisted Intervention (MICCAI) 2019 meeting in Shenzhen, China, a team of researchers led by Wenqi Li of Nvidia shared how they enhanced privacy protections for federated learning -- a method of training AI algorithms using data from multiple institutions without sharing individual site data. In testing, a deep-learning model trained with their federated-learning approach achieved comparable brain tumor segmentation performance to an algorithm that was trained on the entire dataset.
"Data is one of the biggest challenges for [AI], and with privacy-preserving techniques we can potentially enable people to share all of their data without exposing any of it, and be able to create these amazing algorithms that learn from everybody's knowledge," said Abdul Hamid Halabi, global lead for healthcare at Nvidia.
With traditional federated learning, developers can train a deep neural network using training data distributed across multiple sites; each site essentially trains a local model on its own data and then periodically submits the model to a parameter server. This server then aggregates each of these contributions to produce a global model that is then shared with all participating sites. As a result, organizations can collaborate on developing a shared AI model without having to directly share any clinical data, according to the researchers.
Although each site's individual training data are never shared with other sites during federated learning, there are still ways to potentially reconstruct and extract patient data by performing model inversion, according to the researchers. As a result, the group sought to strengthen the privacy and security of federated learning by incorporating selective parameter sharing -- hiding certain parts of the model updates. This concept is implemented with what the researchers call a differential privacy module, which randomly selects the components of the model that are shared with the different sites. This ensures that the updated model that is shared among the different sites does not expose patient data, said co-author Nicola Rieke of Nvidia.
"In the end, we kind of 'distort' the updates, and we limit the information that is shared among the institutions," she told AuntMinnie.com. "In this way, we go one step further with privacy and the federated learning setup for healthcare."
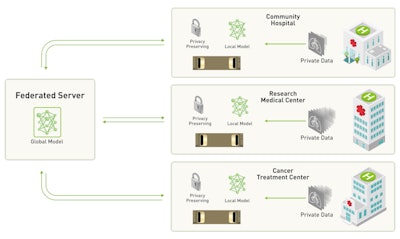
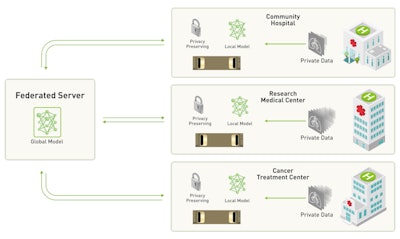
Federated learning concept featuring privacy protection developed by Nvidia and King's College. Image courtesy of Nvidia.
The researchers tested their approach on the Multimodal Brain Tumor Image Segmentation Benchmark (BRATS) 2018 dataset, which includes preoperative multiparametric MRI studies for 285 patients with brain tumors. The dataset includes contributions from 13 different institutions, and the researchers trained and tested the tumor segmentation algorithm using a simulated federated learning approach with the 13 different sites. They also trained the algorithm on the dataset as a whole.
"We investigated for this dataset to see if it was possible to share only 40% of the model ... and we found that we still have the same performance as if all of the data would have been pooled and trained [in a centralized manner]," she said.
Federated learning approaches may also help make AI models more generalizeable by enabling them to be trained on larger and more diverse datasets, she noted. The next step now is to prove this approach further with clinical models and make these tools available for others to use, Halabi said.