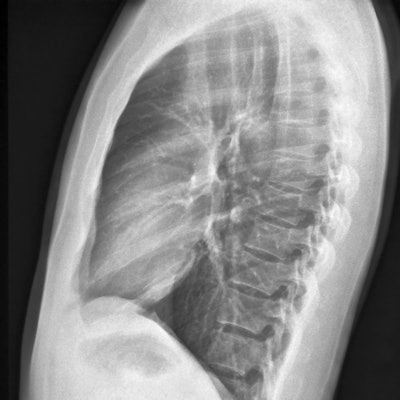
CHICAGO - A deep-learning model that predicts the 10-year risk of death from heart attack or stroke by using a single chest x-ray will be highlighted November 29 at the RSNA annual meeting in Chicago.
A team led by Dr. Jakob Weiss from Massachusetts General Hospital found significant associations between the risk predicted by the model and observed major cardiac events in their study of over 147,000 chest x-rays from more than 40,000 participants.
"As chest radiograph images are commonly available, our approach may help identify individuals at high risk for cardiovascular disease, prompting risk factor assessment and targeted prevention," Weiss and colleagues wrote.
Current guidelines recommend estimating 10-year risk of major adverse cardiovascular disease events to find out who should get a statin to lower cholesterol for disease prevention. Risk scores used for atherosclerotic cardiovascular disease assessment take into account patient age, sex, race, systolic blood pressure measures, hypertension treatment history, smoking status, type 2 diabetes status, and a lipid panel. But these variables may not be available in a patient's electronic record.
Weiss and colleagues wanted to test the potential of artificial intelligence (AI) in estimating 10-year cardiovascular risk from routine chest radiographs. They developed a deep learning model that was trained using 147,497 chest radiographs from 40,643 cancer screening trial participants and conducted independent testing in a separate cohort of 11,430 outpatients who were potentially eligible for intervention.
In the study, major adverse cardiovascular events occurred in 1,096 (9.6%) cases over a median follow-up of 10.3 years. The team found significant ties of risk predicted by the model and major adverse cardiovascular events in patients eligible for statin (hazard ratio [HR], 2.03; 1 is reference). This finding remained significant after adjustment for cardiovascular risk factors (adjusted HR, 1.63).
The researchers also found that in the subgroup where all variables necessary were available to calculate atherosclerotic cardiovascular disease risk, the model showed similar performance and added to the overall risk score (adjusted HR, 1.58).
The team concluded that deep learning could estimate cardiovascular risk from a routine x-ray image similar to the current clinical standard.
"This enables opportunistic screening to identify high-risk patients who would benefit from prevention with statins," Weiss et al wrote in their abstract.