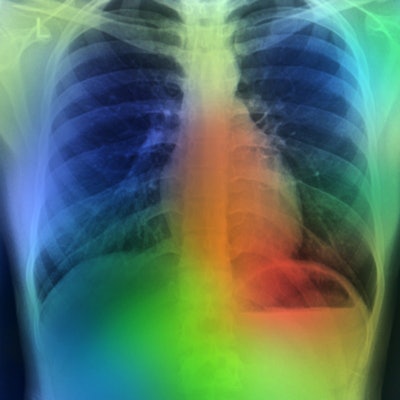
An artificial intelligence (AI) model can identify individuals at risk of death from lung cancer on easily obtainable x-rays, which could improve personalized prevention and treatment strategies, according to a group in Boston.
Researchers led by Dr. Jacob Weiss of Massachusetts General Hospital developed a convolutional neural network (CNN) model to estimate the risk of lung disease mortality using a chest radiograph as the only input. In a test on three datasets comprising more than 15,000 individuals, the model performed well.
"Our findings motivate the use of deep learning to identify individuals at high risk of lung disease mortality from easily obtainable and low-cost chest radiograph images," the group wrote. The study was published May 16 in Nature Communications.
Chest x-ray is a common preliminary test for lung cancer, yet actionable radiological findings are difficult to detect by human readers in the early stages of the disease, the researchers explained. They suggest that AI may provide an early window into the risk and extent of lung disease beyond established methods.
In previous work using chest x-rays, the group has shown that AI can enhance survival predictions in lung cancer based on a patient's biological age. They have also shown that a deep-learning model can predict a patient's 10-year risk of death from heart attack or stroke. In this study, the group developed a CNN they've named CXR-Lung-Risk to identify individuals on x-rays who may be at high risk for lung disease mortality.
The researchers trained the model using 147,497 x-ray images of 40,643 individuals. The images included lung nodules, atelectasis, pleura and lung fibrosis, chronic obstructive pulmonary disease (COPD)/emphysema, opacities, cardiac abnormalities, lymphadenopathy, and bone/chest wall lesions. In addition, data included the dates of actual observed deaths of patients.
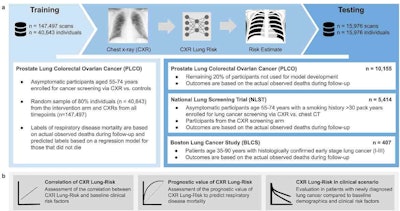
They tested the model in three independent cohorts comprising 15,976 individuals between 35 and 90 years of age enrolled in previous trials: an asymptomatic community population, heavy smokers eligible for lung cancer screening CT exams enrolled in the National Lung Screening Trial (NLST), and patients with histologically confirmed early-stage (I-III) lung cancer.
The researchers noted that risk probabilities are usually expressed in percentages, which can be difficult to grasp. Thus, they devised a method for the model to output risk from a single x-ray expressed in years.
"The only input to the model is a single existing chest radiograph and the output is a risk for lung disease mortality expressed in years, meaning if the model outputs a risk of 75 years this is an equal risk of lung disease mortality as the risk of an average 75-year-old individual," they explained.
After adjusting for risk factors, including age, smoking, and radiologic findings, CXR-Lung-Risk discriminated between individuals at high versus low risk for lung disease mortality, according to the findings. Specifically, the lowest mean CXR-Lung-Risk (63 years) was found on x-rays in the asymptomatic screening population without known lung cancer. Patients in the NLST had a higher CXR-Lung-Risk on average (mean, 66.1 years), while the model estimated the highest mean risk of 70.5 years in patients with histologically confirmed lung cancer.
"We found that CXR Lung-Risk showed a graded association with lung disease mortality after adjustment for risk factors, including age, smoking, and radiologic findings," the group wrote.
Limitations of the study included not knowing which alterations and findings in the x-ray images were important for the model's final prediction, which is a common drawback of deep-learning models, the authors noted. However, association analysis did show correlation with clinical risk factors (e.g., smoking, age, prevalent hypertension) and traditional radiologic findings (e.g., nodules, fibrosis, emphysema), suggesting that the model identifies anatomical changes known to be correlated with increased risk, they added.
"CXR-Lung-Risk could be used with minimal disruption of current clinical workflows and automatically analyze the latest radiograph of a patient at high speed and low additional cost," the group concluded.