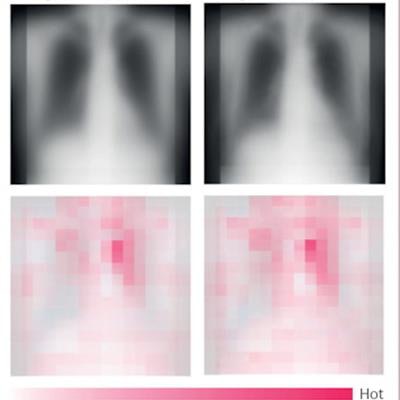
An AI model has been trained to estimate the age of patients from chest x-rays, with findings suggesting that x-rays may help clarify relationships between aging and various diseases, according to a study published August 16 in the Lancet Healthy Longevity.
Researchers led by Yasuhito Mitsuyama, of Osaka Metropolitan University in Japan trained a deep neural network to estimate patient age using more than 100,000 chest x-rays from participants across five institutions. The finding suggests that chest x-ray may serve as a biomarker to assess chronic diseases, the group suggested.
"We created a new biomarker of aging that estimates age from chest radiographs, based on an AI model developed from a cohort of healthy individuals," the group wrote.
As opposed to chronological age (time alive since birth), biological age determinations account for people aging more slowly or rapidly due to unhealthy habits or genetic predispositions. Deep-learning techniques based on imaging have been used previously to establish pediatric bone age, for instance, yet the usefulness of chest x-rays as a biomarker for aging using multi-institutional data remains underexplored, the authors noted.
In this study, the group leveraged deep neural network functions that extract features and learn patterns from x-rays to automatically estimate the age of healthy individuals.
"This is the first multi-institutional study to create and validate a deep learning-based regression model for aging using chest radiographs from healthy participants," the group wrote.
The researchers used 101,296 healthy chest x-rays from 70,248 participants across five institutions. First, they used a dataset from three of the hospitals to train and test a deep neural network called ConvNeXt to identify features that could predict chronological age. On the test dataset, the model showed a correlation coefficient of 0.95 between the AI-estimated age and chronological age, with a coefficient of 0.9 or higher typically considered very strong.
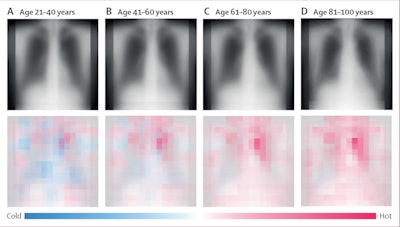
"The AI-estimated age using chest radiographs showed a strong correlation with chronological age in the healthy cohorts," the group wrote.
Importantly, the researchers then tested the model using a separate dataset from two other hospitals that included x-rays of patients with various diseases. They determined whether the diseases were statistically associated with differences between the AI-estimated age and patients' chronological age.
The results revealed that the difference between AI-estimated age and the patient's chronological age was positively correlated with a variety of chronic diseases, such as hypertension, hyperuricemia, and chronic obstructive pulmonary disease.
"In cohorts of individuals with known diseases, the difference between estimated age and chronological age correlated with various chronic diseases," the group noted.
In other words, the higher the AI-estimated age compared to the chronological age, the more likely individuals were to have these diseases, according to the authors.
The findings add to a growing body of literature exploring the concept of estimating biological age from imaging data, the authors wrote. Ultimately, they suggested the use of chest x-ray as a biomarker could pave the way for innovative early diagnostic and preventive approaches toward age-associated diseases.
The full article is available here.