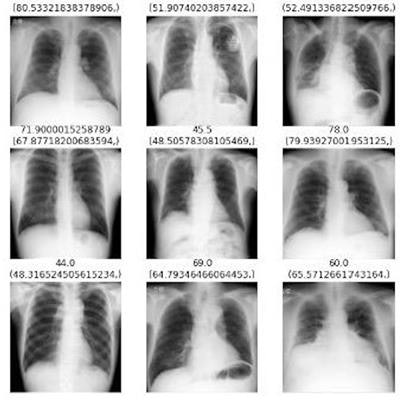
AI can accurately glean height and weight from chest x-rays -- a task that could help clinicians implement rapid nutritional interventions for bedridden patients, according to a group in Nagasaki, Japan.
A team led by Yasuhiko Nakao, MD, PhD, of Nagasaki University, developed a convolutional neural network to predict the height and weight of patients based on more than 14,000 x-rays acquired over a 15-year period. The model's predictions had a high correlation with actual height and weight -- key information for proper nutritional assessment, they wrote.
"Our chest radiographic prediction model has a high correlation with actual height and weight and can be combined with clinical nutrition factor information for rapid assessment of risk for malnutrition," the group noted in a study published September 6 in Clinical Nutrition Open Science.
Current guidelines recommend prompt nutrition intervention and initiation of nutrition therapy within 24 to 48 hours of admission in patients suspected of malnutrition, the authors explained. To determine a patient's metabolic rate and nutrition needs, clinicians use a formula called the Harris-Benedict equation, which requires patients' age, gender, height, and weight. However, determining height and weight can be difficult in patients with serious infections, those in intensive care units, and in bedridden elderly patients with severe contractures, they wrote.
In this study, the researchers aimed to develop a convolutional neural network (CNN) model that could potentially assess height and weight based on chest x-rays. They used 6,453 x-rays from male patients and 7,879 x-rays from female patients to train and test the model, with height and weight data extracted from electronic medical records, then applied an image regression model (ResNet-152) that previously has been reported to predict age from CT imaging data. For input, they prepared linked datasets in which the CNN associated the x-rays with height and weight.
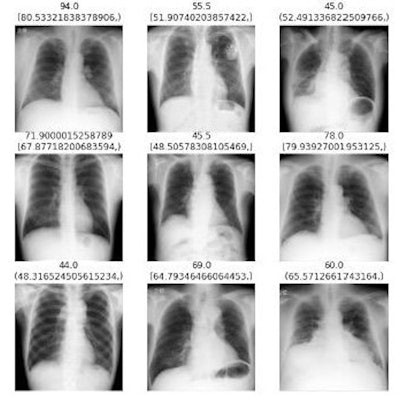
The team based its statistical analysis on the comparison of the model's predictions against the original height and weight values using Pearson's correlation coefficients.
According to the findings, the correlations between the model's predicted values and actual values for males and females were 0.855 and 0.81, while the correlation coefficients for weight for males and females were 0.793 and 0.86.
"Our chest radiographic prediction model has a high correlation with actual height and weight and can be combined with clinical nutrition factor information for rapid assessment of risk for malnutrition," the group wrote.
Ultimately, determining the height and weight of bedridden patients in emergency departments may be delayed during times of high hospital admissions, such as the COVID-19 pandemic, the authors noted. These information delays could be reduced using their model, they suggested.
Also, since the model was developed using standing chest x-rays for which actual height data existed, the authors plan a prospective study in the future comparing the model's rates of agreement based on supine x-rays, they added.
"Importantly, our model is an illustration of the potential of automated imaging AI for proper nutrition prediction models in elderly patients," the researchers concluded.
The full study can be found here.