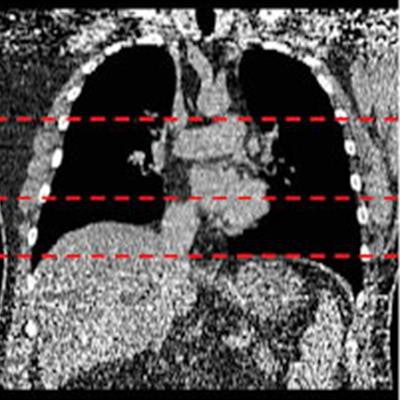
An AI algorithm can opportunistically predict patient mortality risk by analyzing body composition on baseline low-dose CT lung cancer screening exams, according to research published July 25 in Radiology.
A team of researchers led by Kaiwen Xu, a doctoral candidate in the department of computer science at Vanderbilt University in Nashville, TN, tested an internally developed AI algorithm on more than 20,000 patients from the National Lung Screening Tria (NLST). They found that the model could improve performance for predicting lung cancer death, cardiovascular disease death, and all-cause death.
"Automatic AI body composition potentially extends the value of lung screening with low-dose CT beyond the early detection of lung cancer," Xu said in a statement from the RSNA. "It can help us identify high-risk individuals for interventions like physical conditioning or lifestyle modifications, even at a very early stage before the onset of disease."
Having previously developed and tested an AI algorithm to automatically produce body composition measurements on LDCT exams, Xu and colleagues sought in their study to test the value of body composition analysis for predicting incidence of lung cancer.
Of the 20,768 participants included in the study, 865 were diagnosed with lung cancer and 4,180 died during follow-up, according to the researchers. In comparison with Cox proportional hazard models for each specific cause of death, the AI-produced body composition measurements led to better risk prediction – as determined via the likelihood ratio test (expressed as χ2) -- for lung cancer death (male participants: χ2 = 23.09, p < 0.001; female participants: χ2 = 15.04, p = 0.002), cardiovascular disease death (males: χ2 = 69.94, p < 0.001; females: χ2 = 16.60, p < 0.001), and all-cause mortality (males: χ2 = 248.13, p < 0.001; females: χ2 = 94.54, p < 0.001).
It did not, however, improve predictive performance for lung cancer incidence in either male participants (χ2 = 2.53, p = 0.11) or female participants: (χ2 = 1.73, p = 0.19).
In other findings, the researchers noted that measurements associated with fat found within a muscle were particularly strong predictors of mortality. Myosteatosis is now deemed to be more predictive for health outcomes than reduced muscle bulk, according to the authors.
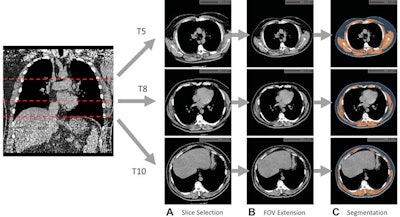
The researchers believe that opportunistic screening has great potential for routine clinical use.
"The images in a CT ordered for quite a different purpose -- in our case, early detection of lung cancer --contain much more information," Xu said. "In the space of the chest CT used for lung cancer screening, you can also check other information like body composition or coronary artery calcification that is directly associated with cardiovascular disease risk."
In the next phase of their work, the researchers would now like to perform a longitudinal study to assess how changes in body composition affect health outcomes.
The complete study can be found here.