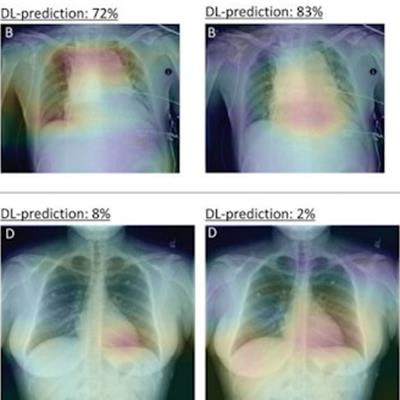
An artificial intelligence (AI) model could help emergency department doctors triage patients with symptoms of acute chest pain syndrome, according to a study published online January 17 in Radiology.
A team at Boston's Massachusetts General Hospital developed an AI deep-learning model that identifies patients with acute chest pain syndrome who are at risk for 30-day acute coronary syndrome, pulmonary embolism (PE), or aortic dissection. In testing, the model improved prediction of these adverse outcomes, they wrote.
"To the best of our knowledge, our deep learning AI model is the first to utilize chest x-rays to identify individuals among acute chest pain patients who need immediate medical attention," wrote lead author Dr. Márton Kolossvary, PhD, and colleagues.
Acute chest pain syndrome accounts for over 7 million emergency department visits annually in the United States. However, fewer than 8% of these patients are diagnosed with the three major cardiovascular causes of acute chest pain syndrome -- acute coronary syndrome, PE, or aortic dissection.
Moreover, the life-threatening nature of these conditions and low specificity of clinical tests, such as electrocardiograms and blood tests, lead to substantial use of cardiovascular and pulmonary diagnostic imaging that can often yield negative results, the authors wrote.
For the study, the team developed and then tested an open-source deep learning model that identifies patients with acute chest pain syndrome who are at risk for acute coronary syndrome, PE, or aortic dissection based on initial chest x-rays.
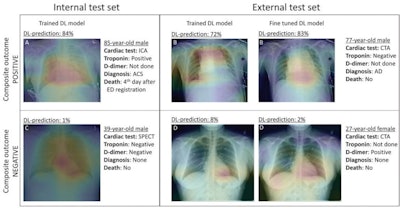
The group used data from adults who presented to the emergency departments of two Boston-area hospitals with acute chest pain syndrome and who underwent chest x-rays, blood tests, and additional cardiovascular or pulmonary imaging or stress testing. The datasets were divided into groups of 17,254 (60%) for training, 5,751 (20%) for validation, and 5,750 (20%) for internal testing.
In testing, the deep-learning tool significantly improved prediction of these adverse outcomes beyond age, sex, and conventional clinical markers, such as D-dimer blood tests, and maintained its diagnostic accuracy across age, sex, ethnicity, and race. Using a 99% sensitivity threshold, the model was able to defer additional testing in 14% of patients as compared with 2% when using a model that only incorporated age, sex, and biomarker data, the researchers reported.
The investigators noted that this was a proof-of-concept study but that in the future, the model could be used to analyze chest x-rays in the background to help select patients who would benefit most from immediate medical attention versus those who may be discharged safely.
Another benefit? Using AI for this indication could lead to significant cost reductions.
"As [emergency departments] struggle with high patient numbers and a shortage of hospital beds, effectively triaging patients with very low risk of acute coronary syndrome, PE, or aortic dissection who would not benefit from additional cardiovascular or pulmonary testing could improve resource and cost efficiency," the authors concluded.