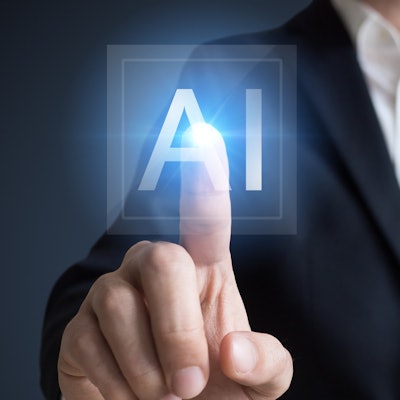
A wide range of claims are advertised by developers of AI for neuroimaging, yet insufficient data have been published publicly to support these claims, according to researchers at Yale University in New Haven, CT.
A team led by Suryansh Bajaj, MD, scoured the websites of AI developers to analyze trends in U.S. Food and Drug Administration (FDA)-cleared algorithms for neuroimaging and to understand their advertised value propositions. The group found the cart may be leading the horse.
"Further research is necessary to determine whether the value claimed by the developer is actually demonstrated in clinical practice," the authors wrote.
Funding for companies developing medical imaging AI applications has totaled almost $3.5 billion since 2015, according to the authors, with neuroradiology leading subspecialties in terms of the number of algorithms cleared by the FDA. Given this rapid growth, it is important to understand the different applications and the value these software tools provide in clinical practice, they wrote.
In the first of a series of planned studies, the group looked into the proposed value propositions of FDA-cleared algorithms in neuroimaging.
The group extracted a list of AI algorithms for neuroimaging from the American College of Radiology (ACR) Data Science Institute AI Central database. They collected product information for each device, including information on the advertised value as presented on the developer's website.
According to the findings, a total of 59 AI neuroimaging algorithms were cleared by the FDA between May 2008 and August 2022. Most of these algorithms (24/59) were compatible with noncontrast CT, 21 with MRI, nine with CT perfusion, eight with CT angiography, three with MR perfusion, and two with PET. Six algorithms were compatible with multiple imaging techniques.
The following are the advertised value proposition for these algorithms:
- Improved quality of care (38/55, 69.1%)
- Saving user time (24/55, 43.6%)
- Decreased costs (9/55, 15.7%)
- Increased revenue (6/55, 10.9%)
"Despite all these proposed benefits and value propositions offered by the AI/[machine learning]-driven devices, there are certain challenges when it comes to their implementation in daily practice," the group wrote.
To wit, "there is frequently a lack of publicly available data supporting these claims," the authors wrote.
While such data is made available to the FDA, it is often not transparently available to the public and vendors before or at the time of purchase of the product, they added.
Another area of concern regards costs. The researchers found that 88% (52/59) of the algorithms were FDA-cleared for single-use cases with binary outcomes (the presence or absence of intracranial hemorrhage, for instance). The practical integration of such AI algorithms into clinical radiology workflows would likely require the implementation of several products to ensure a reasonable breadth of triage of critical findings, they suggested.
"The total financial burden of this extent of integration often remains hidden due to the overt marketing of these devices," the group wrote.
Ultimately, the researchers encouraged continued efforts by radiologic organizations to make AI more mainstream and said that the study highlights the need for improved standardization and transparency of advertised AI value propositions.
The full article is available here.