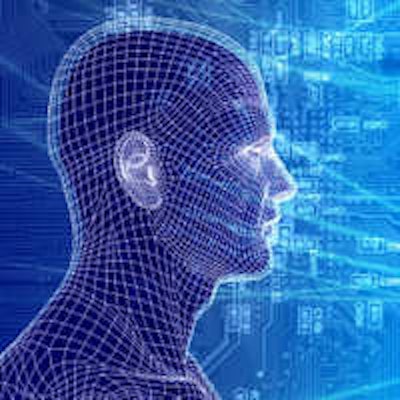
Are radiologists really "wasted protoplasms" -- as allegedly described recently by a CEO of a medical imaging start-up company -- who can be easily replaced in the future with artificial intelligence (AI) technology? Not so fast, according to Dr. Eliot Siegel of the University of Maryland.
Despite a number of predictions that it won't be long before computers obviate the need for radiologists, vast technical and even regulatory barriers will prevent that from happening for a very long time.
"I believe that imaging is likely to be the ultimate frontier [for AI], rather than the first thing to fall in medicine," he said. "You shouldn't have any fear of being replaced by computers, at least in radiology, anytime soon."
Machine learning and AI technology do offer much potential for improving medicine and diagnostic imaging in the short term, however, Siegel said. He shared his predictions for the future role of AI during the Sam Dwyer Lecture at the closing session of the recent Society for Imaging Informatics in Medicine (SIIM) annual meeting in Portland, OR.
Current status
What would Dwyer -- widely considered to have been a PACS pioneer -- have thought about AI and its potential in diagnostic imaging?
"I think Sam would be surprised that computer-aided detection and diagnosis hasn't really made as much progress as I bet he would have anticipated," Siegel said. "We have tens of thousands of machine-learning algorithms, but almost no connection between these research algorithms and their clinical application. There have been relatively small, incremental improvements over the years in some of these analytic algorithms."
There's been much hype lately and concern over the potential "threat" of machine learning and AI technology to radiologists. In fact, Siegel dedicated his talk to a first-year radiology resident from Portugal who recently emailed him to see if he would continue to recommend radiology as a career in light of the recent developments in AI.
While there have been incredible advances over the past few years in object recognition technology, they don't necessarily apply to radiology.
"I see no evidence whatsoever to suggest at this point that there's any fundamental change in our ability to apply even these convolutional neural networks in the radiology space to get to the point where we can 'replace the findings made by radiologists,' " Siegel said.
Human vision is one of the hardest tasks for computers, he added.
One of the problems is that object recognition algorithms are designed for very specific purposes and haven't been tested on the grayscale images used in radiology. In addition, these algorithms are good at identifying that an object is a chair, but they can't tell if the chair is broken, if something is missing or extra, or if the chair is beautiful or ugly, or dirty or clean, Siegel said.
"At this point, those tasks that might be analogous to what we do in radiology are well beyond what anybody is able to do with the training on convolutional networks," he said.
Black box
There's also the "black box" aspect of these algorithms, as they can't explain why an object has been identified as abnormal.
Siegel also repeated his offer that he would go anywhere in the world to wash the car of anybody who can show him an algorithm that can identify the adrenal gland as well as he can teach a fifth grader to do in 10 minutes.
"I've been repeating that [challenge] for 15 years, and I still haven't washed any cars yet," he said. "If a computer in 2016 can't even find the adrenal glands, then I'm not sure how they're going to replace me as a radiologist, or anybody else."
There's no general-purpose learning system for diagnostic imaging that exists like we train radiology residents, according to Siegel.
"Our eyes and brains have evolved over many, many years to detect patterns and combine that with our knowledge of medicine, physiology, a priori likelihood of disease, and [recognition of trends]," he said.
There are a number of difficult challenges in using machine-learning algorithms in radiology, including deciding on which algorithm to use, figuring out how to evaluate different machine-learning algorithms, and determining which algorithm is the most efficient for a particular problem.
Specific machine-learning algorithms do a good job at different tasks, which makes it difficult to select a single one as a generalized approach for image analysis or for data analysis. In addition, electronic medical records (EMRs) have hundreds of dimensions that require different approaches.
"We don't have any techniques or machine-learning techniques that can get at solving those types of challenges," Siegel said.
Slow adoption of CAD
The history of computer-aided detection (CAD) software in radiology demonstrates the challenges of implementing this type of technology. Although reports of CAD software began appearing in the literature in 1990, mammography CAD still isn't trusted by most radiologists, according to Siegel.
The problem with CAD is that it's very much a black box, he said.
"Either a lesion gets circled or it doesn't," he said. "If I had a resident who refused to tell me why they were circling the lesion or how confident they were, I would be very suspicious and frustrated with them."
Siegel noted that there have been tens of thousands of algorithms developed for image analysis and decision support over the past 30 years. Hardly any are in clinical practice.
"In order to replace a radiologist, you would have to find the best of these [algorithms] and consolidate them into a package that would work independently [for image review]," he said.
These algorithms are also written in different "languages" with different assumptions, and they have a narrow focus. To replace a radiologist, there would have to be a general portfolio of algorithms that could perform as well as specialists currently do.
"The work on computer vision that can recognize a water bottle in an image database is fundamentally different than the type of data that we have in diagnostic imaging, and no one has any evidence of any algorithm or methodology that could be comparable to what's being used for the imaging challenges [in diagnostic radiology]," Siegel said.
What's more, assuming this was all available and somehow integrated, it would need to go through the U.S. Food and Drug Administration (FDA) approval process. It could take up to decades to get approval for each and every one of those algorithms.
Even if a program was discovered or created that was so "smart" that it could read textbooks and journal articles and could review prior images on PACS and prior reports in the EMR, and it was better than any subspecialty radiologists at all tasks, "how would you test it and how long would it take to figure out that it was actually better than any subspecialty radiologist?" Siegel said. "Would it take years or decades to be able to figure that out? We barely know how to test humans [in these areas], much less computers."
Other issues include the time and cost to get the datasets to train the algorithms. These algorithms would also raise significant medicolegal issues, such as knowing when algorithms could be trusted.
"It's much more likely that we'll see continued incremental changes [from machine-learning algorithms]," Siegel said.
Exciting potential
Machine-learning algorithms do offer exciting potential for many radiology applications, however. Siegel said he would like to see learning algorithms help with areas such as the following:
- Intelligent screening criteria for mammography, lung cancer, and other cancers
- Patients at risk for contrast reactions
- Automatic protocoling of studies
- Smart PACS hanging protocols and synchronization protocols
- Smart transfer of findings from workstations to speech recognition software
- Assessment of patients who have high risk (or low risk) of positive findings
- Communication and tracking of findings
- Multiparametric analysis across multiple modalities
- Improved departmental efficiency with decreased waiting times
- Dose optimization
- Quality improvement in scanning
Siegel closed with a message for the radiology resident from Portugal who had written him for advice on whether he should continue with his radiology training.
"Please finish your residency," he said. "There's lots of work to do before we can have a general machine-learning, deep-learning, and AI program that can learn radiology conceptually and rapidly like a radiology resident."
Siegel said there are a number of vendors who have great ideas in this area, and there is much "low-hanging fruit" that could be great targets for machine-learning techniques.
"There are so many amazing things that we can do with advanced statistics and deep learning," he said. "Let's do those now and forget about the silliness with being worried about replacing the radiologist."