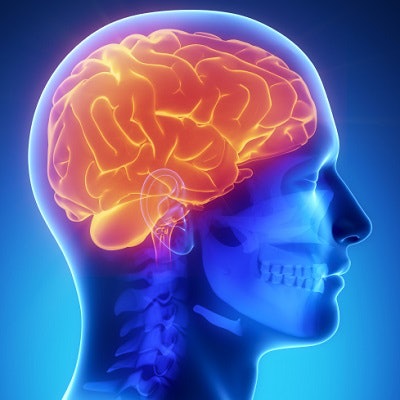
Can machine learning be used to diagnose serious cases of depression based on data from MRI scans in adults, potentially even before they begin showing symptoms? Yes, it can, according to a proof-of-concept study being published in the June issue of Psychiatry Research: Neuroimaging.
Researchers from Texas trained a machine-learning algorithm to analyze brain white-matter measurements derived from MRI scans of adults with major depressive disorder (MDD), as well as from healthy individuals. They found that the algorithm produced 74% accuracy in classifying the study participants.
Importantly, the group also learned that neuroimaging information that can predict depression is distributed across several brain networks, rather than being localized in a single network of the brain.
"The results of our machine-learning classification hold the promise of predicting who is depressed or even potentially who might be vulnerable to depression," wrote the study team, which was led by cognitive neuroscientist David Schnyer, PhD, from the University of Texas at Austin (Psychiatry Res, June 30, 2017, Vol. 264, pp. 1-9).
The power to predict?
While researchers have previously studied the use of brain MRI to diagnose mental disorders, most of those neuroimaging projects have been descriptive in nature rather than predictive, according to the authors.
"The brain networks may appear to differ between two groups [of patients], but it doesn't tell us about what patterns actually predict which group you will fall into," Schnyer said in a news release from the Texas Advanced Computing Center (TACC) in Austin, where the group has been developing and training its machine-learning algorithm using the center's Stampede supercomputer. "We're looking for diagnostic measures that are predictive for outcomes like vulnerability to depression or dementia."
The group set out to explore the clinical utility of diffusion-tensor MRI (DTI-MRI) for classifying individuals diagnosed with major depressive disorder. Schnyer and colleagues also wanted to explore the predictive power of support vector machines (SVMs), a machine-learning technique.
After recruiting 45 healthy controls and 52 participants seeking treatment for MDD, they also selected a subset of 25 healthy control patients and 25 individuals with MDD who were matched for age and gender on a 1:1 basis. All patients in this group ranged in age from 18 to 35.
The participants were imaged on a 3-tesla whole-body MRI scanner (Signa Excite, GE Healthcare) with an eight-channel head coil. White-matter measurements were derived from a high angular resolution diffusion imaging (HARDI) MR sequence that was collected using single-shot echo-planar imaging and a twice-refocused spin-echo pulse sequence that was optimized to minimize eddy current-induced distortions, according to the researchers.
Fractional anisotropy measurements
After comparing the fractional anisotropy measurements of the DTI-MRI exams, the researchers found statistically significant differences between the two groups of participants in the study. They then reduced the number of voxels involved to a subset that was most relevant for classification, and used the machine-learning approach to perform classification and prediction.
The algorithm performed best when only right hemisphere white matter was examined, yielding 68% sensitivity, 80% specificity, and classification accuracy of 74%.
The results show that DTI-derived fractional anisotropy maps can accurately classify depressed or vulnerable individuals versus healthy controls, according to the researchers. They also noted that classification accuracy was aided by white-matter fractional anisotropy values in elements across all three brain networks previously associated with major depressive disorder, rather than being highly localized.
"Not only are we learning that we can classify depressed versus nondepressed people using DTI data, we are also learning something about how depression is represented within the brain," senior author Christopher Beevers, PhD, said in the TACC statement. "Rather than trying to find the area that is disrupted in depression, we are learning that alterations across a number of networks contribute to the classification of depression."
As a result, it likely isn't possible to accurately classify MDD based on using one white-matter tract or brain network, according to the researchers.
"Fortunately, advances in machine learning and related statistical techniques allow for the integration of highly dimensional data into prediction algorithms," they wrote. "These methods therefore appear to have substantial promise for the development of diagnostic tools that can objectively classify the presence or absence of major depressive disorder and other psychiatric disorders."
The group acknowledged that the predictive power of the team's machine-learning method now must be demonstrated on new and independent datasets.
Adding more data
In the next phase of their work, the researchers hope to improve the performance of machine-learning algorithms by incorporating more data, not just from MRI scans, but also from genomics and other relevant classifiers, according to the TACC. They will also expand their study to include data from several hundred volunteers in the Austin community who have been diagnosed with depression, anxiety, or a related condition.
The group will also utilize Stampede 2 -- the TACC's new supercomputer that will come online later in 2017. It will be twice as powerful as the original Stampede, according to the center.