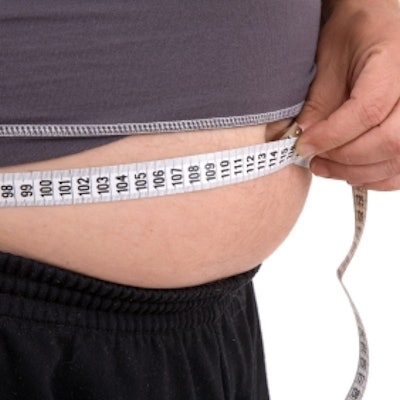
Artificial intelligence (AI) algorithms can enable screening for metabolic syndrome in individuals receiving abdominal CT exams for other reasons and who don't have symptoms of this dangerous condition, according to research published online November 10 in the American Journal of Roentgenology.
A team of researchers led by first author Dr. Perry Pickhardt of the University of Wisconsin School of Medicine and Public Health in Madison, WI, and senior author Dr. Ronald Summers, PhD, of the U.S. National Institutes of Health (NIH) Clinical Center in Bethesda, MD, found that automated algorithms for quantifying fat, muscle, and liver on low-dose unenhanced abdominal CT scans performed during colorectal cancer screening could also be helpful for identifying patients at risk for metabolic syndrome.
"These measures can be opportunistically applied to CT scans obtained for other clinical indications, possibly leading to unsuspected detection and potential intervention of this important yet reversible condition," the authors wrote. "Perhaps even more importantly, utilizing these CT-based visceral measures could potentially lead to a more relevant and fundamental definition of the metabolic syndrome."
To evaluate the use of automated CT-based measures of body composition for identification of metabolic syndrome in adults without symptoms, the researchers retrospectively applied International Diabetes Federation criteria for diagnosing metabolic syndrome to 7,785 adults who had received low-dose, unenhanced abdominal CT for routine colorectal cancer screening between 2004 and 2016 at their institution.
Of these patients, 738 (9.5%) met the criteria for metabolic syndrome, and subsequent major cardiovascular events occurred significantly more frequently in this group of patients (p < 0.001). Next, the CT exams of all 7,785 patients were analyzed by image-processing and deep-learning algorithms previously developed at the University of Wisconsin for quantifying muscle, visceral and subcutaneous fat, liver fat, and abdominal calcification.
The researchers found a significant difference for all abdominal CT-based biomarker measures between patients with metabolic syndrome and those who did not have the syndrome (p < 0.001).
Performance of abdominal CT-based biomarkers for diagnosing metabolic syndrome | ||||
Abdominal aortic calcification scoring | Volumetric liver attenuation | Skeletal muscle index | L1-level total adipose tissue | |
Area under the curve | 0.578 | 0.738 | 0.776 | 0.909 |
Odds ratio | 1.6 | 5.1 | 5.8 | 27.2 |
In other findings, the researchers found that in women, the algorithms produced an area under the curve of 0.93 when the measurements of skeletal muscle index and total adipose tissue were combined. Also, a total abdominal fat threshold of 460.6 cm² yielded 80.1% sensitivity and 85.4% specificity for metabolic syndrome.
The findings parallel other studies that have found that data acquired in CT screening exams can be used for purposes other than the original exam, such as using CT lung screening exams to calculate bone density.
In an accompanying editorial comment on the current study, Dr. Orlando Catalano of the Varelli Diagnostic Institute in Naples, Italy, said that the study results imply that these algorithms may help alert asymptomatic subjects receiving CT scans for a variety of reasons of their risk for metabolic syndrome.
"At the same time, CT measures could be used to improve our knowledge of the multifaceted connections between body composition and metabolic and cardiovascular disease," Catalano wrote.
Catalano encourages the authors to also consider other potential applications for their techniques.
"For example, in patients with cancer who undergo serial CT examinations, it would be quite interesting to better understand the evolution of sarcopenia and cachexia over time," Catalano wrote.