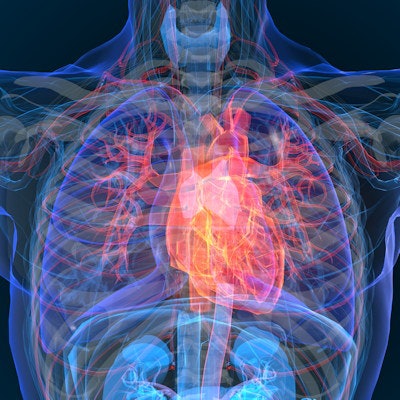
Combining machine learning with coronary CT angiography (cCTA) to assess cardiovascular health may improve clinicians' ability to predict a patient's risk of future adverse events like unstable angina, according to a presentation delivered at the recent American Roentgen Ray Society (ARRS) meeting.
The findings are good news for a vulnerable population of patients -- and their physicians, wrote a team led by presenter Dr. Verena Brandt of Robert-Bosch-Hospital in Stuttgart, Germany.
"Machine learning-based cCTA-derived plaque quantification and characterization may have utility in risk-stratifying the vulnerability of coronary lesions for the prediction of future major adverse cardiac events," the researchers wrote.
Time-consuming
Previous studies have shown that cCTA-based risk scores can help predict whether patients are vulnerable to unstable angina -- and therefore heart attack -- or even cardiac death, Brandt said. These scores reflect coronary plaque burden by location and extent, as well as the severity of coronary artery disease. But the process of evaluating this clinical data can be time-consuming. Machine learning offers a time-efficient way to do this, Brandt said.
The researchers conducted a study that included 361 patients with suspected coronary artery disease who underwent cCTA; they then noted any major adverse cardiac events that occurred within 90 days after the exam. Brandt's team used a machine-learning algorithm trained on cCTA-derived plaque measures, conventional CT risk scores, and cardiovascular risk factors to predict adverse outcomes, measuring its performance using the area under the receiver operating characteristic curve (AUC).
Plaque measures included the following:
- Low-attenuation plaque
- Napkin ring sign
- Remodeling Index
- Segment stenosis score
- Segment involvement score
- Spotty calcifications
Of the total patient cohort, 31 (8.6%) experienced a major adverse cardiac event. The algorithm showed higher predictive power than conventional CT risk scores, plaque measures, and regression analysis.
Performance of machine-learning algorithm compared with other methods/data for predicting adverse cardiac events | |
Measure | AUC |
Machine-learning algorithm | 0.96 |
Conventional regression analysis | 0.94 |
High-risk plaque features | 0.89 |
Segment stenosis score | 0.89 |
Agatston calcium score | 0.84 |
Cardiovascular risk factors | 0.83 |
Obstructive coronary artery disease | 0.79 |
Machine learning could improve the care and management of patients at risk for serious heart conditions, according to Brandt.
"Machine learning may improve the integration of patients' [clinical] information to improve risk stratification," she concluded.
Calcium quantification
In a related presentation, a team led by presenter Dr. Vincent Giovagnoli of the Medical University of South Carolina in Charleston investigated the performance of a deep learning-based automated calcium quantification algorithm of chest and cardiac CTs. The algorithm was trained on 1,261 dedicated cardiac-CTs and further refined on 500 nongated chest-CTs; its performance was compared with Agatston scores from cardiac-CTs, and manually determined calcium volume and clinical calcium classifications (none, mild, moderate, and severe) data from chest-CTs.
The study included 95 patients who underwent both dedicated cardiac and chest CT, with a median number of days between the two exams of 186. These patients were compared with a control group of 168 people who underwent chest CT qualitative calcium classification only.
The AI algorithm performed well compared with all the other modes of assessment:
- Agatston score and AI-determined calcium volume showed a correlation of 0.92.
- Manual calcium volume assessment of all 95 patients showed a correlation with the algorithm of 0.92.
- The algorithm showed good agreement with clinical qualitative analysis, at 0.74.
As the adverse cardiac events research suggested, deep learning could improve the management of at-risk patients, according to the group.
"Deep-learning-based calcium quantification on chest-CTs shows good correlation compared to both quantitative and qualitative reference standards," Giovagnoli and colleagues concluded. "Fully automating this process may reduce evaluation time and optimize clinical calcium scoring without additional acquisitions. AI-based calcium quantification on chest CTs might aid in the evaluation of cardiovascular risk, especially in a screening setting."