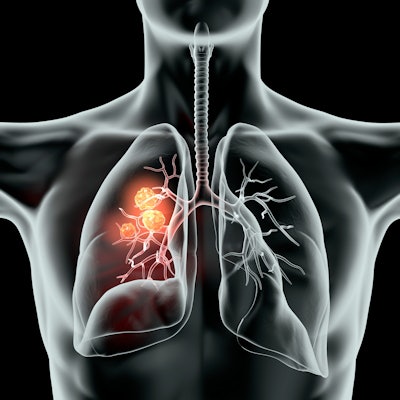
An artificial intelligence (AI) algorithm can predict lung cancer subtypes based on F-18 FDG-PET/CT imaging, potentially offering advantages over biopsies in these patients, according to research published recently in Academic Radiology.
A group led by Dr. Hongyue Zhao from The First Affiliated Hospital of Harbin Medical University in Harbin, China, trained a deep-learning model on 189 preoperative F-18 FDG-PET/CT images from patients with non-small cell lung cancer (NSCLC). In testing, the model performed well in distinguishing between the two most common forms of NSCLC: lung adenocarcinoma (ADC) and lung squamous cell carcinoma (SqCC).
"In many cases, doctors must make subjective judgments on the pathological subtypes of NSCLC by combining laboratory markers and imaging findings," the authors wrote. "Consequently, rapid and noninvasive methods of distinguishing lung ADC from lung SqCC are crucial for therapeutic strategies for precision medicine."
Differentiating between the two main subtypes of NSCLC is crucial; drugs developed for lung ADC are generally ineffective against lung SqCC, the authors explained. Increasing evidence suggests that F-18 FDG-PET can provide quantitative tumor information to identify these NSCLC subtypes, yet few studies have combined this data with deep learning, the authors wrote. To that end, the group performed an exploratory study to investigate seven deep-learning algorithms to distinguish histological subtypes in patients with NSCLC.
The researchers collected preoperative F-18 FDG-PET/CT images of 189 patients with NSCLC. Of these, 82 patients were diagnosed with SqCC and 107 cases had ADC. Using 1,280 features extracted from the images, the researchers trained and optimized seven deep-learning algorithms: Shufflenet, VGG16, Googlenet, Inception v3, Resnet50, Densenet201, and Mobilenet v2.
The Mobilenet v2 model achieved the best overall performance. On an internal test dataset of 38 PET/CT fused images, the model distinguished NSCLC subtypes with an area under the receiver operating characteristic curve (AUC) of 0.744. What's more, it produced an AUC of 0.767 on an "extended" test cohort of 38 patients that included tumor segmentations performed by a nuclear medicine physician. As a result, the model showed good generalizability and reproducibility, according to the researchers.
Ultimately, as an exploratory study, the research was limited by a small sample size, yet it represents a step forward in noninvasive detection of pathological subtypes of NSCLC, the authors noted.
"Importantly, this study demonstrated the value of the [deep-learning] techniques in discriminating pathological subtypes of NSCLC from preoperative F-18 FDG-PET/CT images," the group concluded.